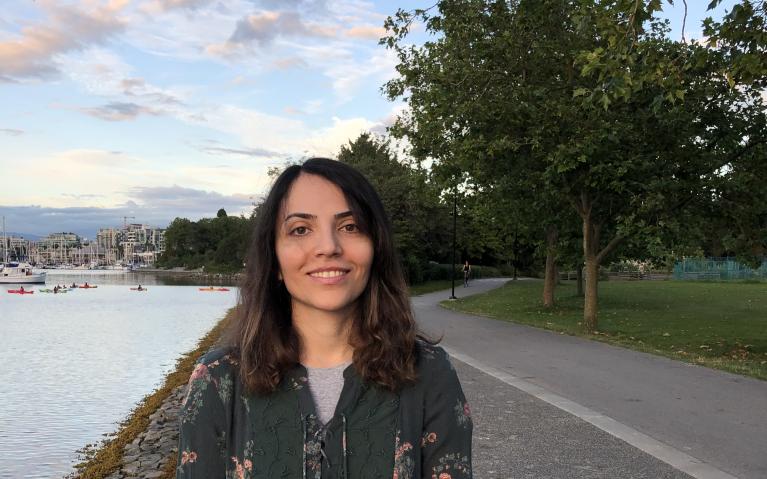
Faeze Keshavarz
Doctor of Philosophy in Bioinformatics (PhD)
Research Topic
Understanding cellular pathways modifications in cancer using the transcriptome
Bioinformatics Cancer Genomics
G+PS regularly provides virtual sessions that focus on admission requirements and procedures and tips how to improve your application.
These videos contain some general advice from faculty across UBC on finding and reaching out to a potential thesis supervisor.
Dissertations completed in 2010 or later are listed below. Please note that there is a 6-12 month delay to add the latest dissertations.
Genome sequencing has transformed our understanding of human genetic diseases in recent years, not least of which is Cancer. Among the genetic abnormalities commonly observed within cancer are copy number variants, alterations in the abundance of DNA, which often affect cellular function and contribute to disease. Whole-genome sequencing has allowed for high throughput examination and identification of mutations such as single nucleotide variants within cancer, while the identification of copy number variants remains comparatively difficult. The critical task for accurate identification of copy number variants from this data remains segmentation, the task of aggregating sequences of DNA abundance observations into contiguous segments of presumably constant DNA copy number. In this dissertation, we propose a novel method for performing copy number segmentation of sequenced whole cancer genomes. We apply a novel bottom-up, coarse-to-fine segmentation algorithm alongside statistical techniques to identify tumor heterogeneity and accurately perform copy number variant detection. We compare our method with a number of other methods in a variety of contexts, including fully synthetic data, resequenced cell line data, and a large cohort of sequenced metastatic cancer genomes. Next, we apply the results of our method in the analysis of chromosomal instability patterns throughout the genome. We assess genome-wide patterns of homozygous deletion and methods of measuring chromosomal instability and its numerous interfaces with tumor biology, including mutational signatures and gene dosage effects. Finally, we investigate the prospect of identifying copy number variants using long read data from oxford nanopore instruments. We present two case reports of copy number variation analysis in these data, and subsequently assess our ability to identify these variants in metastatic cancer biopsies as compared to traditional short read sequencing methods. We subsequently investigate factors influencing our ability to identify copy number events in nanopore data. In this work, we have focused on the methods and analysis of copy number variants in human cancer. The methods and analyses performed herein will assist in research concerning these mutations and their greater role in cancer and human biology.
View record
DNA cytosine methylation is the most common epigenetic mark of the mammalian genome. This epigenetic modification is involved in different biological processes and its aberrations are involved in various disorders. While it is a well-studied epigenetic mark, the limitations of array-based and short-read technologies to detect DNA methylation have resulted in a gap in the study of allele-specific methylation and DNA methylation at repetitive genomic regions. Nanopore sequencing offers long-read sequencing and detection of both DNA bases and modification simultaneously. Therefore, offers the potential to overcome the caveats of previous technologies. In this thesis, I aimed to investigate genome-wide DNA methylation from nanopore sequence data and develop tools and workflows for the detection of allelic methylation using this technology and study genome-wide allele-specific methylation and imprinting. Moreover, I aimed to leverage long-read data and their methylation information to develop a novel approach for improved and parent-of-origin-aware phasing. I explored DNA methylation detection using nanopore sequencing and demonstrated that nanopore DNA methylation data are highly correlated with current widely used approaches. To detect allele-specific methylation using nanopore data, I developed the NanoMethPhase tool and workflow that enables detection of allele-specific methylation genome-wide. Using NanoMethPhase and nanopore sequencing data for several normal human cell lines, I explored genome-wide allele specific methylation and detected 42 novel imprinted regions and 7 large blocks of imprinted methylation. Using a combination of nanopore sequencing and strand-seq with methylation at human imprinted regions, I developed a novel methodology that enables the assignment of genomic variants to their parental origin without any data from parents. Finally, I used nanopore sequencing to study DNA methylation in advanced tumour samples from the personalized onco-genomics program at BC Cancer. I detected several hundred genes across tumour samples with tumour-specific allelic methylation and aberrant expression. I further detected several known imprinted regions with loss of imprinted methylation across tumour samples. Through these projects and studies, I developed tools and methods that enable allele-specific methylation analysis and parent-of-origin-aware genomic phasing. Moreover, I contributed to our understanding of allele-specific methylation and imprinting in normal and cancerous cells.
View record
Cancers are characterized by somatic mutation arising from the interplay of mutagen exposure and deficient DNA repair. Whole genome sequencing of tumours reveals characteristic patterns of mutation, known as mutation signatures, which often correspond with specific processes such as cigarette smoke exposure or the loss of a DNA repair pathway. Quantifying DNA repair deficiency can have clinical implications. Cancer chemotherapies which induce DNA damage are known to be more effective against cancers with deficient DNA repair. However, it is not yet known whether mutation signatures can serve as reliable predictive biomarkers for response to these treatments. Furthermore, the current understanding of mutation signatures stems largely from studies of primary, untreated tumours, whereas metastasis underpins as much as 90% of cancer-related mortality. This thesis aims to (1) describe the association between mutation signatures and clinical response to DNA damaging chemotherapy, (2) enable accurate personalized assessment of mutation signatures and their evolution over time, and (3) characterize the evolution of mutational processes in metastatic cancers. To assess clinical actionability, we quantified signatures of single nucleotide variants, structural variants, copy number variants, and small deletions in 93 metastatic breast cancers, 33 of which received platinum-based chemotherapy. We found that patients with signatures of homologous recombination deficiency had improved responses and prolonged treatment durations on platinum-based chemotherapy. Next, we formulated a Bayesian model called SignIT, which improves the accuracy of individualized mutation signature analysis and infers signature evolution over tumour subpopulations. We demonstrated SignIT’s superior accuracy on both simulated data and somatic mutations from The Cancer Genome Atlas, and validated temporal dissection using whole genomes from 24 multiply-sequenced cancers. We highlighted a potential clinical application of mutation in a BRCA1-mutated pancreatic adenocarcinoma with low Homologous Recombination Deficiency (HRD) signature but exceptional response to platinumcontaining chemotherapy. Finally, we deciphered mutation signatures from nearly 500 metastatic cancer whole genomes, revealing evolution of mutational processes associated with late metastasis and exposure to cytotoxic chemotherapy. Taken together, our findings demonstrate the complex interplay of factors shaping the metastatic cancer genome. We highlight both clinical opportunities of studying genomic instability and the additional insights available from understanding their temporal evolution.
View record
The highest number of cancer-associated deaths are attributable to metastasis. These include rare cancer types that lack established treatment guidelines, or cancers that become resistant to established lines of therapy. Precision oncology projects aim to develop treatment options for these patients by obtaining a detailed molecular view of the cancer. Scientists use sequencing data like whole-genome sequencing and RNA-sequencing to understand the biology of the cancer. A significant challenge in this process is diagnosing the cancer type of the sample since the observed measurements are best understood with this context. Routine histopathology relies on tissue morphology and can fail to provide a determinative diagnosis when the cancer metastasizes, presents biology attributable to multiple different cancer types, or presents as a rare cancer type. Molecular data has revealed differences in the genetic makeup of cancers that appear morphologically similar, motivating the use of molecular diagnostics. Nevertheless, no existing tools utilize the output from these sequencing modalities in its entirety (that is, without feature selection). There is also limited work evaluating the utility of pan-cancer molecular diagnostics in a precision oncology trial. In this work we review an ongoing precision oncology trial and identify the impact of sequencing-based approaches on cancer diagnosis. We develop SCOPE, a machine-learning method that uses RNA-Seq profiles of tumours for automated cancer diagnosis. We show that this method, which uses over 17,688 gene measurements as input, has better classification accuracy than when using statistically prioritized marker genes, can deconvolve cancer-types with mixed histology, and has high performance in metastatic cancers and cancers of unknown origin. In precision oncology, manual analysis of the tumour's genomic profile is used to understand tumour biology and driver pathways. We find that by assessing the classifier's dependence on gene subsets, we can automatically calculate the importance of various biological programs in individual tumours. Pathways prioritized through this tool - called PIE - show a high overlap with manual integrative analysis performed by expert bioinformaticians to identify clinically important genomic changes. Lastly, we demonstrate that PIE facilitates cohort-wide cancer analysis and discovery of novel sub-groups in advanced cancers.
View record
Biomedical researchers have the overwhelming task of keeping abreast of the latest research. This is especially true in the field of personalized cancer medicine where knowledge from different areas such as clinical trials, preclinical studies, and basic science research needs to be combined. We propose that automated text mining methods should become a commonplace tool for researchers to help them locate relevant research, assimilate it quickly and collate for hypothesis generation. To move towards this goal, we focus on extracting relations from published abstracts and full-text papers. We first explore the use of co-occurrences in sentences and develop a method for inferring new co-occurrences that can be used for hypothesis generation. We next explore more advanced relation extraction methods by developing a supervised learning method, VERSE, which won part of the BioNLP 2016 Shared Task. Our classical method outperforms a deep learning method showing its applicability to text mining problems with limited training data. We develop it further into the Kindred Python package which integrates with other biomedical text mining resources and is easily applied to other biomedical problems. Finally, we examine the applicability of these methods in personalized cancer research. The specific role of genes in different cancer types as drivers, oncogenes, and tumor suppressors is essential information when interpreting an individual cancer genome. We built CancerMine, a high-quality knowledgebase, using the Kindred classifier and annotations from a team of annotators. This allows for quantifiable comparisons of different cancer types based on the importance of different genes. The clinical relevance of cancer mutations is generally locked in the raw text of literature and was the focus of the CIViCmine project. As a collaboration with the Clinical Interpretation of Variants in Cancer (CIViC) project team, we built methods to prioritise relevant papers for curation. Through this work, we have focussed on different ways to extract structured knowledge from individual sentences in biomedical publications. The methods, guidelines, and results developed will aid biomedical text mining research and the personalized cancer treatment community.
View record
Discovering biomarkers and molecular drivers of head and neck endocrine tumors was the inspiration for this thesis. Here, I describe the molecular evaluation of tumors of the thyroid and parathyroid endocrine glands for the purpose of identifying somatic driver alterations in these cancers. While molecular interplay of the germline genomic background of an individual and the somatic genome that emerges throughout the lifetime plays significant roles in increasing the susceptibility to cancer and in driving the malignant phenotype, the major known contributors to cancer remain the acquired somatic mutations. Analysis of a sporadic and recurring parathyroid carcinoma, with incidence of 1 per million population, revealed mutations in mTOR, MLL2, CDKN2C and PIK3CA and comparison of patient-matched primary and recurrent malignant tumors uncovered loss of PIK3CA activating mutation during the evolution of the tumor. Loss of the short arm of chromosome 1 along with somatic missense and truncating mutations in CDKN2C and THRAP3 provided new evidence for the potential role of these as tumor suppressors. Hürthle cell thyroid carcinoma accounts for a small proportion of all thyroid cancers; however, this malignancy often presents at an advanced stage and poses unique challenges. Genomic analysis revealed large regions of copy number variation encompassing nearly the entire genomes accompanied also by near haploidization. Moreover, I identified loss-of-function mutations of the tumor suppressor gene MEN1 in 4% of patients. Repeated alterations of the epigenetic machinery in anaplastic thyroid carcinoma, one of the most fatal of all adult solid malignancies, and novel gene fusions including MKRN1-BRAF, FGFR2-OGDH and SS18-SLC5A11 are reported here. The transcriptomic analysis suggested known drug targets such as FGFRs, VEGFRs, KIT and RET to have low expressions in this cancer; however, through integrative data analysis, I identified the mTOR signaling pathway as a potential therapeutic target for anaplastic thyroid cancer. Molecular analysis of papillary thyroid carcinoma and benign thyroid nodules revealed very low mutation rates in these tumors with CYP1B1, PTPRE, CTSH and RUNX1 emerging as promising diagnostic markers. The key somatic mutations identified in these studies can serve as novel diagnostic markers as well as therapeutic targets.
View record
Next Generation Sequencing (NGS) technologies enable Deoxyribonucleic Acid (DNA) or Ribonucleic Acid (RNA) sequencing to be done at volumes and speeds several orders of magnitude faster than Sanger (dideoxy termination) based methods and have enabled the development of novel experiment types that would not have been practical before the advent of the NGS-based machines. The dramatically increased throughput of these new protocols requires significant changes to the algorithms used to process and analyze the results. In this thesis, I present novel algorithms used for Chromatin Immunoprecipitation and Sequencing (ChIP-Seq) as well as the structures required and challenges faced for working with Single Nucleotide Variations (SNVs) across a large collection of samples, and finally, I present the results obtained when performing an NGS based analysis of eight mammary ductal carcinoma cell lines and four matched normal cell lines.
View record
Repositioning existing drugs for new therapeutic uses is an efficient approach to drugdiscovery. However, most successful repositioning cases to date have been serendipitous; thegoal of my thesis was to use computational methods to rationally discover drug repositioningcandidates.I first virtually screened (VS) 4621 drugs against 252 drug targets with molecular docking.This method emphasized removing potential false positives using stringent criteria fromknown interaction docking, consensus scores, and rank information. Published literatureindicated experimental evidence for 31 top predicted interactions, supporting the approach.The chemotherapeutic nilotinib was validated as a potent MAPK14 inhibitor in vitro (IC5040nM), suggesting a potential use in inflammatory diseases.I then applied this method to the cancer target EGFR, predicting the anti-HIV drug tenofovirdisoproxil fumarate (TDF) as a novel inhibitor. In vitro, TDF inhibited the proliferation andEGFR-signaling of an EGFR-overexpressing cell line, but did not inhibit EGFR in directkinase binding assays. This study highlighted limitations of computational and experimentalmethodologies that should be considered when interpreting or designing other studies.We then screened 1,120 off-patent drugs against the triple-negative breast cancer (TNBC)target p90RSK using both VS and high-throughput (HTS) methods. VS predicted a set ofcompounds 26-times enriched for known RSK inhibitors and 11 times enriched for HTS hits,underscoring its efficiency. In secondary screens, the chemotherapeutic ellipticine and thebioflavonoids luteolin and apigenin inhibited RSK activity (IC50 0.50-4.77μM), blocked RSKsignaling, and inhibited TNBC cell proliferation. These drugs thus have potential to berepositioned to TNBC.Finally, we rationally repositioned renal cell carcinoma drugs for a patient with a rare tongueadenocarcinoma. Whole genome and transcriptome sequencing of the patient’s tumor andnormal cells detected sequence, copy number, and expression aberrations, and analysis suggested that the tumor was driven by the RET oncogene. Treatment with RET-inhibitingdrugs stabilized the disease for eight months, after which the disease progressed. We alsosequenced the post-treatment tumor and found changes consistent with acquired therapeuticresistance.Overall, this thesis details two novel high-throughput approaches for drug repositioning:virtual screening of drugs and targets and personalized medicine via sequencing.
View record
No abstract available.
Theses completed in 2010 or later are listed below. Please note that there is a 6-12 month delay to add the latest theses.
Cancer genomes often carry somatic mutations that disrupt gene expression on specific alleles, leading to Allele-Specific Expression (ASE). ASE may dysregulate cancer driver genes by favoring loss-of-function alleles, causing haploinsufficiency. However, detecting ASE requires precise genomic variant phasing, a challenge with previous phasing methods such as pseudo-phasing algorithms, short-read phasing and population phasing. These methods are limited by low accuracy, small phase blocks and inability to phase rare and somatic variants.Our objective is to develop and validate a bioinformatics pipeline for genome-wide ASE gene detection by integrating Nanopore long-read sequencing for precise genomic variant phasing with short-read RNA-sequencing for enhanced ASE detection. We will conduct an exploratory analysis of ASE data using a pan-cancer cohort as part of the Personalized OncoGenomic (POG) project. Our aim is to identify the genomic mechanisms responsible for genes exhibiting ASE, explore known instances of ASE, and utilize ASE to identify and validate dysregulated cancer genes that may contribute to tumor development.To achieve these goals, we have developed IMPALA, a pipeline designed for the detection of ASE genes and the identification of potential genetic mechanisms behind ASE. Additionally, we have developed a novel ASE simulator capable of generating synthetic RNA-seq data containing known ASE genes. Using the simulated data, IMPALA demonstrates an average F1 score of 0.93. ASE analysis was done on 179 tumor samples from the POG cohort, where an average of 26% of phased genes exhibited ASE.We explore various cis-acting genetic mechanisms that can lead to ASE, including allelic CNV imbalance, allelic promoter methylation silencing, and nonsense-mediated decay. Notably, we explored known instances of ASE, such as X-inactivation genes, imprinting genes, and their correlation with allelic methylation of imprinting control regions. Furthermore, we employ ASE to investigate clinically relevant cancer genes, focusing on somatic mutations found on the major expressing allele. Leveraging ASE, we identify 631 significant noncoding mutations linked to the expression of cancer genes, potentially serving as markers for cis-acting regulatory mechanisms. Lastly, we delve into the specific abnormal ASE of cancer genes, such as DUSP22, to uncover potential genetic mechanisms behind these ASE events.
View record
N⁶-methyladenosine (m6A), a prevalent internal methylation pattern in mammalian mRNA, plays a pivotal role in various aspects of RNA biology. m6A modification has been implicated in the regulation of hematopoiesis and hematologic malignancies, particularly in the context of Acute Myeloid Leukemia (AML). However, the comprehensive understanding of m6A distribution and stoichiometry at a single-base resolution, along with its interaction with other RNA features, remains limited. Existing methodologies for m6A mapping face challenges in providing single-nucleotide resolution, creating a gap in our understanding of m6A variation within transcripts and its interconnectedness with other post-transcriptional regulatory mechanisms. To address these limitations, we employed nanopore direct RNA sequencing on the myeloid leukemia cell line MOLM13, obtaining a comprehensive and transcriptome-wide profiling of m6A stoichiometry and polyA tail lengths at the single-molecule level. Our study focused on the impact on the modifications and correlation with other RNA features when the m6A writer METTL3 is depleted, demonstrating its role in post-transcriptional regulations and their interplay within full-length mRNA molecules in AML cells. METTL3 deletion led to alterations in m6A levels, polyA tail lengths, and transcript expression patterns. Overall, this study provides a foundation for further investigations into the epitranscriptomic landscape of AML and opens avenues for targeted therapeutic interventions.
View record
Structural variants (SVs) are classified as large scale DNA modifications exceeding 50 base pairs. Despite their relatively low abundance, they can play a large functional role in the progression of diseases like cancer. Traditionally, SVs have been studied using short reads sequencing, but this approach limits by the length of reads, and misses out on events such as large insertions. In cancer, specific SVs are clinically actionable, and thus is imperative to accurately detect them. This thesis aims to assess read-based SV callers, aligners, and reference genomes for long reads to establish best practices, investigate methods for reducing false positives in SV detection by integrating various reference genomes and tools, and illustrates the benefits of SV calling with long reads using the Personalized OncoGenomics (POG) cohort. Key findings include the necessity of achieving a minimum coverage of 15X for accurate SV event detection in germline samples, underscoring the importance of employing an ensemble SV calling approach to capture diverse signals. We demonstrate the ability of long reads to enhance resolution for complex SV events overlooked by Illumina sequencing, illustrated by examples involving predicted pathogenic cancer genes like SMG1 and HIRA. The study confirms biological literature findings, such as high insertion signals in microsatellite instable tumours and unique inversion counts indicative of a unique signal of SVs, “tyfonas”. However, challenges like coverage persist, leading to false negatives. The Nanopore POG dataset holds promise for future SV calling software development. Overall, the research highlights the crucial role of long-read sequencing in somatic SV calling in cancer research, emphasizing the intricacies involved in accurately characterizing SV events in tumour genomes.
View record
The work contained within this thesis sought to accurately classify 55 primary cancer subtypes, 20 metastatic cancer subtypes, and 16 normal tissues using gene expression data. The classification was done using a multiple learning task approach in which an artificial neural network model makes four distinct classifications at varying levels of biological hierarchy for each input sample. These learning tasks were the organ system of origin, the disease state, the cancer type, and the cancer subtype. The model achieved classification performance ranging from a macro F1-score of 0.987 within the disease state learning task to 0.831 within the cancer subtype learning task on a test set composed of primary cancer, metastatic cancer, and normal tissue samples. Having shown good classification performance of the model, the second part of the thesis focused on leveraging what the model has learned to extract biological information about the various cancers present in the data set. A backpropagation-based tool called DeepLift was used to generate a list of importance scores for each gene within every class of each learning task. The list of scores was then analyzed for trends that could be utilized to infer biological insight about specific cancer types and subtypes, and between primary and metastatic cancers as individual groups. The lists provide a means to functionally annotate enriched pathways and to quantify and compare the role of RNA genes and pseudogenes across various classes and learning tasks. Some of the results output by DeepLift were validated for their biological relevance by presenting supporting evidence from relevant scientific literature. The ultimate product of this thesis research is a tool with which one can quantify the role of a variety of genes within cancers spanning both primary and metastatic cancer types. Further analysis of the output generated by the tool could provide a better understanding of the role of genetic expression, including RNA and pseudogenes, within a variety of different cancers.
View record
Cancer is a disease which arises due to somatic alterations in the genome. However, most studies on cancer genetics only explore the impact that coding mutations have on the progression of the disease. Furthermore, many genomic inquiries on cancer only implicate primary untreated tumours, which misses the impact of metastasis and treatment. Here we present a cohort of 638 advanced cancer patients with whole genomic, transcriptomic and clinical information. Through this cohort, we attempt to better characterize the non-coding region of metastatic cancers as well as attempt to understand the mutational impact of chemotherapeutics. Using a positional clustering method, we identified 1,567 significant mutational hotspots in the genome. 86 genes were identified as being affected by a hotspot in a regulatory region, including in the TERT promoter, a region with well-known driving mutations. To characterize the biological function of the hotspots, we analyzed the impact of mutation on corresponding gene expression. We show an increased expression for TERT and AP2A1 when their respective promoter regions are mutated, the latter being a novel association. Mutational clusters affecting non-coding RNAs were also examined for any functional impact, but no significant associations were seen. Large non-coding mutational events such as kataegis were seen in multiple cancer types and across all chromosomes. However, little recurrence was seen for kataegis. Additionally, using observed mutational frequencies, we attempt to identify any mutations that may be treatment-induced. Examining the breast, lung, colon and pancreas and ovarian cohorts, we were able to extract known resistance mutations such as ESR1 mutations after aromatase inhibitor treatment and EGFR T790M mutations post anti-EGFR therapy. Further insights are required to confirm the expressional change seen in the cohort. Additional studies to determine AP2A1’s role in cancer would help understand this correlation. Overall, our study shows the presence of important mutations in the non-coding space of metastatic cancers, and the power of whole genome sequencing. Furthermore, we display the need for similar datasets to extrapolate mutations which correlate to resistance.
View record
Personalized medicine approaches for cancer therapy seek to determine optimal therapies for cancer patients based on the molecular profile of their tumour. The motivation is to target oncogenomic alterations in tumours with the appropriate therapies. However, it is currently infeasible to determine the optimal therapy simply given the genomic profile of a tumour. There has been significant recent work in attempting to use the computational approach of machine learning for predicting tumour drug response. Machine learning methods have been successfully used for drug response prediction in cancer cell lines and even have been extended to predicting individual cancer patient response to a small number of chemotherapies. This work uses support vector machines (SVM) to predict the response to chemotherapies of 570 advanced cancer patients from the BC Cancer Personalized OncoGenomics program using the transcriptomic profile of their tumours. This dataset of advanced cancers presents over 20 cancer types and 130 unique chemotherapies. F-measures for the SVM predictions were found to be as high as 1.0 for some cohorts. Further analysis on the set of important genes for the SVMs revealed biological explanations that may explain the SVM predictions. This work demonstrates the value of large-scale sequencing projects and the potential of data mining and machine learning in personalized cancer medicine.
View record
Pancreatic ductal adenocarcinoma (PDAC) is considered the most lethal common cancer, with the highest incidence-to-mortality ratio of any solid tumour. Molecular pathology studies and genomic analyses have improved our understandings of how PDAC develops and progresses, and there has been significant progress in treatment strategies for specific genomic alterations. One of these alterations is the NRG1 gene fusion, which has been found to be a rare, but potentially targetable oncogenic driver. To determine whether PDAC patients have an NRG1 gene fusion, we used convolutional neural networks (CNNs) to analyze digital whole slide images (WSIs) of cancer biopsies. In particular, we used histopathological H&E slides from the Personalized OncoGenomics program to train a deep CNN (VGG-16) framework that automatically classifies normal tissue, NRG1-fusion positive tumour tissue, and NRG1-fusion negative tumour tissue. We implemented the model in two-stages, where the first stage classifies normal from tumour tissue, and the second stage classifies the tumour tissue as being NRG1-fusion positive or negative. The model achieved accuracies of 86.5% and 76.0% for each stage, respectively, and an overall accuracy of 68.8%. Additionally, we found that PDAC cases with high expression of the NRG1 gene (93rd-98th percentile of TCGA PDAC cases) were being classified as NRG1-fusion positive, suggesting a possible correlation between NRG1 gene fusions and high parent gene expression. Finally, we attempted to understand the inner workings and decisions of our CNN model by analyzing internal feature maps. We found activation patterns that matched distinct histological features and compared them with a more traditional image segmentation approach. Overall, our findings demonstrate that deep CNNs have the potential to assist pathologists in detecting therapeutically actionable genomic markers.
View record
Genomic sequencing technology provides insight into cancer pathogenesis and tumoural mechanisms. Tumour RNA sequencing can be used to assess the functionality of genes by allowing for gene expression quantification and transcriptome analysis. Mutational signatures are somatic patterns of mutations arising from specific mutagenic processes such as exogenous and endogenous exposures, defective DNA repair mechanisms or DNA enzymatic editing. Such signatures are “genomic scars” informing on the underlying biological processes that led to cancer. Whole genome sequencing (WGS) of tumour DNA and matched blood DNA as well as whole transcriptome sequencing (WTS) of tumour RNA was performed in advanced cancers of diverse types as part of the Personalized OncoGenomics project. Germline single nucleotide variants (SNVs), copy number variants (CNVs) and structural variants (SVs) in 98 hereditary cancer genes were analyzed from germline WGS data. Somatic SNVs, CNVs and SVs were analyzed from tumour WGS and WTS data. Somatic SNVs profiles were used for mutational signature modelling. Gene expression was obtained from WTS. Transcriptome targeted assembly was performed for transcript splicing analysis. We present specific examples demonstrating the usefulness of combined genomic and bioinformatic approaches for understanding clinically unusual cases of cancer and their molecular mechanisms. We used somatic mutational signature profiling to determine the functional impact of germline and somatic variants in MUTYH, a base excision repair gene, on the overall mutational landscape. In Chapter 2, we present a case series of patients with germline MUTYH variants and diverse cancers. We identified two MUTYH variants for which the previous classification in public databases are inconsistent and we show that these variants cause aberrant splicing and base excision repair deficiency signatures enriched for C:G>A:T transversion mutations. Our results support the pathogenicity of these variants. In Chapter 3, we present the example of comprehensive genomic profiling of a rare and uncharacterized tumour, the eccrine porocarcinoma, in which CDKN2A was identified as a potential novel driver. In both chapters, we used transcriptome targeted assembly to detect and characterize aberrant splicing due to selected germline and somatic variants of interest.
View record
The thyroid gland, necessary for normal human growth and development, is essential for the regulation of metabolism. Its function – to produce and secrete appropriate levels of thyroid hormone – is simple; however accurate assessment of thyroid abnormality is challenging and a fundamental understanding of the normal thyroid is therefore needed. One way to characterize the normal functioning of the thyroid gland is to study the epigenome and resulting transcriptome within its constituent cells. In this study, we compare the consistency of chromatin state annotations across the epigenomes from the grossly uninvolved tumour-adjacent thyroid tissue of four human individuals using ChIP-seq and RNA-seq. We profile four activating (H3K4me1, H3K4me3, H3K27ac, H3K36me3) and two repressing (H3K9me3, H3K27me3) histone modifications, identify chromatin states using a hidden Markov model, produce a novel metric for model selection, and establish epigenomic maps of 19 chromatin states. We found that epigenetic features characterizing promoters and transcription elongation tend to more consistent across epigenomes and that epigenetically active genes consistent across all epigenomes tend to have higher expression than those that are not marked as epigenetically active in all samples. We also identified a set of 18 genes epigenetically active and consistently expressed in the thyroid that are likely relevant to thyroid function. Altogether, we believe the epigenomes presented in this work represent a useful resource to gain a deeper understanding of the underlying molecular biology of thyroid function and provide contextual information of thyroid and human epigenomic data for comparison and integration into future studies.
View record
Retrieving relevant scientific papers in a scalable way is increasingly important, as more and more studies are published. PubMed’s relevant article recommendation is based on MeSH assignments by indexers, which requires significant human resources and can become a limitation in making papers searchable. Many recommendation systems use singular value decomposition (SVD) to pre-compute related products. In this study, we look at using latent semantic analysis (LSA), an application of SVD to determine relationships in a set of documents and terms, to find related biomedical papers. We focused on determining the best parameters for SVD in retrieving relevant biomedical articles given a paper of interest. Using PubMed's recommendations as guidance, we found that using cosine distance to measure document similarity leads to better results than using Euclidean distance. We re-evaluated other parameters, including the weighting scheme and the number of singular values and using a larger abstract corpus. Finally, we asked people to compare the relevant abstract retrieved with our method against those retrieved by PubMed. Our method retrieved sensible articles that were chosen over PubMed's relevant papers one-third of the time. We looked into the abstracts retrieved by either method and discuss possible areas for experimentation and improvement.
View record
The alignment of short DNA read sequencing data to a human reference genome sequence has become a standard step in the analysis pipeline for short DNA read sequence data. As the rate at which short read DNA sequence data is being produced doubles every 5 months, analysis of this data in a computationally efficient way is becoming increasingly important.We demonstrate how we can exploit the ``embarrassingly parallel'' property of short read sequence alignment in custom-designed hardware in FPGA’s. Hardware is chosen, a system is designed, and this system is implemented.My FPGA-based hit finder was demonstrated to produce correct hit results. The performance of this single FPGA implementation was demonstrated to be 71,000 seed hits found per hour on a human genome sized reference sequence. The implementation was demonstrated to produce identical results to the hit finder stage of the MAQ aligner.We demonstrate that the price/performance of this sliding-window FPGA aligner (approximately ~355 seeds/hr/$) compares favorably to the price/performance of sliding-window software aligners (approximately ~67.5 seeds/hr/$ for MAQ). However, software aligners which are based on the superior Burrows-Wheeler alignment algorithm still have a significant price/performance advantage over the FPGA-based approach (approximately ~7,200 seeds/hr/$). We predict that as chips continue to increase in size due to Moore’s Law and computation is performed in high-density cloud-computing datacenters the FPGA-based approach will become preferable to current software aligners.
View record
The immune system plays a critical role in cancer prevention and development. The stimulation of natural immune reaction in a cancer patient by adoptive T-cell therapy has shown success in treating metastatic melanomas and renal cell carcinomas. However, the use of adoptive T-cell therapy remains limited due to unpredictable outcomes and low response rates. In particular, adoptive T-cell therapy for breast cancer has not been realized, despite of the presence of immunogenic antigens such as over-expressed HER2, present in 20-40% of breast tumours. Using a unique transgenic mouse model, the global profiles of gene expression, miRNA abundance and single nucleotide variants (SNVs) were investigated to identify the molecular difference of murine mammary tumours with isogenic background, which exhibited complete regression (CR), partial regression (PR) or progressive disease (PD) outcome of adoptive T-cell therapy. The bioinformatics analyses were further carried out to identify uniquely activated pathways, prognostic gene expression signatures, the effect of post-transcriptional gene regulation and mutated genes unique to tumours with specific outcome. The largest differences in gene expression, miRNA and SNV profiles were repeatedly observed between the regressing (CR, PR) and non-regressing (PD) tumours, supporting the attribution of molecular differences to the immunotherapy outcome. In particular, the gene expression signatures derived from genes in immune-related pathways were experimentally validated to be strong prognostic markers for predicting the CR outcome. Comparison with the human breast cancer subtypes further revealed similarities of the non-regressing tumours with the basal subtype, and the regressing tumours with the HER2 subtype. The difference in miRNA profiles between CR and PR tumours suggested potential translational activities unique to PR, which was nearly identical to CR at the transcriptome level. The findings from this study show that tumour-derivied factors that either promote or suppress the immune system are responsible for the varying outcome of immunotherapy, and that the molecular characteristics can be further applied for the development of clinical prognostic tools, cancer vaccines and drug targets to enhance the efficacy of adoptive T-cell therapy.
View record
If this is your researcher profile you can log in to the Faculty & Staff portal to update your details and provide recruitment preferences.