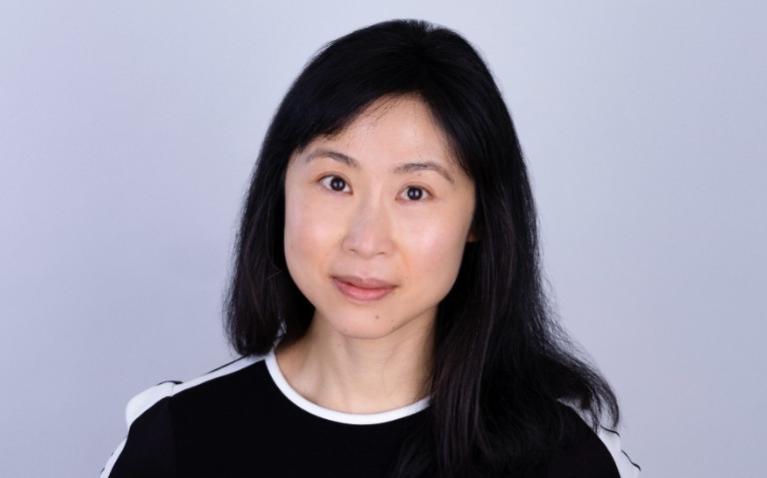
Betty Shea
Doctor of Philosophy in Computer Science (PhD)
Research Topic
Optimization methods
Dissertations completed in 2010 or later are listed below. Please note that there is a 6-12 month delay to add the latest dissertations.
Optimization and inference are essential ingredients of machine learning (ML). Many optimization and inference problems can be formulated from a probabilistic perspective to exploit the Fisher-Rao geometric structure of a probability family. By leveraging the structure, optimization and inference methods can improve their performance. A classic approach to exploiting the Fisher-Rao structure is natural-gradient descent (NGD). However, implementing NGD remains computationally intensive. When a parameter space is constrained, it can also be non-trivial to perform NGD while taking the constraint into consideration. It is even more challenging to perform NGD in structured parameter spaces. This work pushes the boundary of the theory and practice of NGD. We first establish a connection among NGD, mirror descent (MD), and Riemannian gradient descent (RGD). Thanks to this connection, we consider various approaches to better exploit underlying geometric and algebraic structures of NGD resulting in computationally efficient and practically useful methods for inference and optimization problems in ML. In the first part of this work, we reduce the computational cost of NGD for exponential family approximations in variational inference. To do so, we exploit a convex duality in MD by viewing NGD as a MD update.Secondly, we extend the scope of NGD and develop efficient methods by exploiting a similar duality for mixtures of exponential family approximations. Mixtures are more powerful than the exponential family to model complex distributions. We introduce a new Fisher-Rao metric for a mixture since the original Fisher-Rao metric becomes singular in mixture cases. The third part of this work addresses the constraint issue in NGD by proposing a new efficient update scheme inspired by RGD. We focus on performing NGD updates in positive-definite constrained parameter spaces as these spaces appear in exponential family distributions and their mixtures. Finally, we enable a practical usage of NGD methods in structured and constrained parameter spaces by using tools from Riemannian geometry. We propose a structure-preserving NGD update scheme by using matrix Lie groups and moving coordinates. Thanks to our approach, we alsodevelop novel structured second-order methods for unconstrained optimization and adaptive gradient-based methods for deep learning.
View record
Algorithm designers are regularly faced with the tedious task of finding suitabledefault values for the parameters that impact the performance of algorithms. Thoroughlyevaluating even a single parameter configuration typically requires runningthe algorithm on a large number of problem instances, which can make the processvery slow. To address this problem, many automated algorithm configurationprocedures have been proposed. The vast majority of these are based on powerfulmeta-heuristics with strong diversification mechanisms, thereby ensuring that theysufficiently explore the parameter configuration space.However, despite the prominence of automated algorithm configuration, relativelylittle is known about the algorithm configuration landscapes searched bythese procedures, which relate parameter values to algorithm performance. As aresult, while these strong diversification mechanisms make existing configuratorsrobust, it is unclear whether or not they are actually required or simply increase therunning time of the configurators.One particularly notable early work in the field showed evidence suggestingthat the algorithm configuration landscapes of two optimization algorithms are, infact, close to uni-modal. However, existing fitness landscape analysis techniquesare unable to account for the stochasticity in the performance measurements ofalgorithms in a statistically principled way, which is a major barrier to their applicationto studying algorithm configuration scenarios. We address this gap bydeveloping the first statistically principled method for detecting significant deviationsfrom uni-modality in a stochastic landscape.We apply this method, along with other (new and existing) landscape analysistechniques, to a variety of algorithm configuration scenarios arising in automated machine learning (AutoML) and the minimization of the running time ofalgorithms for solving NP-hard problems. We show that algorithm configurationlandscapes are most often highly structured and relatively simple.Using the intuition from this analysis, we develop two prototype algorithmconfiguration procedures designed for AutoML. We show that the methods makeassumptions that are too strong, leading to mixed results. However, we build onthis intuition and develop another procedure for the configuration of NP-hard algorithms.Compared to state-of-the-art baselines, we show that our new methodoften finds similar or better configurations in the same or less time.
View record
Nucleic acid molecules are vital constituents of living beings. These molecules arealso utilized for building autonomous nanoscale devices with biological and technologicalapplications, such as toehold switches, algorithmic structures, robots,and logic gates. Predicting the kinetics (non-equilibrium dynamics) of interactingnucleic acid strands, such as hairpin opening and strand displacement reactions,would assist with understanding the functionality of nucleic acids in the cell andwith building nucleic-acid based devices.Continuous-time Markov chains (CTMC) are commonly used to predict thekinetics of these reactions. However, predicting kinetics with CTMC models ischallenging. Because, first, the CTMCs should be defined with accurate and biophysicallyrealistic kinetic models. Second, the state space of the CTMCs may belarge, making predictions time-consuming, particularly for reactions that happenon a long time scale (rare events), such as strand displacement at room temperature.We introduce an Arrhenius kinetic model of interacting nucleic acid strandsthat relates the activation energy of a state transition with the immediate local environmentof the affected base pair. Our model can be used in stochastic simulationsto estimate kinetic properties and is consistent with existing thermodynamic modelsthat make equilibrium predictions. We infer the model’s parameters on a widerange of reactions by using mean first passage time (MFPT) estimates. We estimateMFPTs using exact computations on simplified state spaces. We show thatour new model surpasses the performance of the previously established Metropoliskinetic model.We further address MFPT estimation and the rapid evaluation of perturbed parameters for parameter inference in the full state space of reactions’ CTMCs.We show how to use a reduced variance stochastic simulation algorithm (RVSSA)to estimate MFPTs. We also introduce a fixed path ensemble inference (FPEI)approach for the rapid evaluation of perturbed parameters. These methods arepromising, but they are not suitable for rare events. Thus, we introduce the pathwayelaboration method, a time-efficient and probabilistic truncated-based approach foraddressing both mentioned tasks. We demonstrate the effectiveness of our methodsby conducting computational experiments on nucleic acid kinetics measurementsthat cover a wide range of rates for different type of reactions.
View record
Deep neural networks have dominated performance benchmarks on numerous machine learning tasks. These models now power the core technology of a growing list of products such as Google Search, Google Translate, Apple Siri, and even Snapchat, to mention a few. We first address two challenges in the real-world applications of deep neural networks in computer vision: data scarcity and prediction reliability. We present a new approach to data collection through synthetic data via video games that is cost-effective and can produce high-quality labelled training data on a large scale. We validate the effectiveness of synthetic data on multiple problems through cross-dataset evaluation and simple adaptive techniques. We also examine the reliability of neural network predictions in computer vision problems and show that these models are fragile on out-of-distribution test data. Motivated by statistical learning theory, we argue that it is necessary to detect out-of-distribution samples before relying on the predictions. To facilitate the development of reliable out-of-distribution sample detectors, we present a less biased evaluation framework. Using our framework, we thoroughly evaluate over ten methods from data mining, deep learning, and Bayesian methods. We show that on real-world problems, none of the evaluated methods can reliably certify a prediction. Finally, we explore the applications of deep neural networks on high-resolution portrait production pipelines. We introduce AutoPortrait, a pipeline that performs professional-grade colour-correction, portrait cropping, and portrait retouching in under two seconds. We release the first large scale professional retouching dataset.
View record
This work considers optimization methods for large-scale machine learning (ML).Optimization in ML is a crucial ingredient in the training stage of ML models.Optimization methods in this setting need to have cheap iteration cost. First-ordermethods are known to have reasonably low iteration costs. A notable recent classof stochastic first-order methods leverage variance reduction techniques to improvetheir convergence speed. This group includes stochastic average gradient(SAG), stochastic variance reduced gradient (SVRG), and stochastic average gradientam´elior´e (SAGA). The SAG and SAGA approach to variance reduction useadditional memory in their algorithm. SVRG, on the other hand, does not needadditional memory but requires occasional full-gradient evaluation. We first introducevariants of SVRG that require fewer gradient evaluations. We then presentthe first linearly convergent stochastic gradient method to train conditional randomfields (CRFs) using SAG. Our method addresses the memory issues requiredfor SAG and proposes a better non-uniform sampling (NUS) technique. The thirdpart of this work extends the applicability of SAGA to Riemannian manifolds.We modify SAGA with operations existing in the manifold to improve the convergencespeed of SAGA in these new spaces. Finally, we consider the convergence ofclassic stochastic gradient methods, based on mirror descent (MD), in non-convexsetting. We analyse the MD with more general divergence function and show itsapplication for variational inference models.
View record
In 2012, deep learning made a major comeback. Deep learning started breaking records in many machine learning benchmarks, especially those in the field of computer vision. By integrating deep learning, machine learning methods have became more practical for many applications like object counting, detection, or segmentation. Unfortunately, in the typical supervised learning setting, deep learning methods might require a lot of labeled data that are costly to acquire. For instance, in the case of acquiring segmentation labels, the annotator has to label each pixel in order to draw a mask over each object and get the background regions. In fact, each image in the CityScapes dataset took around 1.5 hours to label. Further, to achieve high accuracy, we might need millions of such images. In this work, we propose four weakly supervised methods. They only require labels that are cheap to collect, yet they perform well in practice. We devised an experimental setup for each proposed method. In the first setup, the model needs to learn to count objects from point annotations. In the second setup, the model needs to learn to segment objects from point annotations. In the third setup, the model needs to segment objects from image level annotations. In the final setup, the model needs to learn to detect objects using counts only. For each of these setups the proposed method achieves state-of-the-art results in its respective benchmark. Interestingly, our methods are not much worse than fully supervised methods. This is despite their training labels being significantly cheaper to acquire than for the fully supervised case. In fact, in fixing the time budget for collecting annotations, our models performed much better than fully supervised methods. This suggests that carefully designed models can effectively learn from data labeled with low human effort.
View record
Recent years have witnessed huge advances in machine learning (ML) and its applications, especially in image, speech, and language applications. Optimization in ML is a key ingredient in both the training and hyperparameter tuning steps, and it also influences the test phase. In this thesis, we make improvements to optimization of all of these three problems. The first part of the thesis considers the training problem. We present the first linearly-convergent stochastic gradient method to train conditional random fields (CRFs) using the stochastic average gradient (SAG) method. Our method addresses the memory issues required for SAG and proposes a better non-uniform sampling (NUS) technique. The second part of the thesis deals with memory-free linearly-convergent stochastic gradient method for training ML models. We consider the stochastic variance reduced gradient (SVRG) algorithm, which normally requires occasional full-gradient evaluations. We show that we can decrease the number of gradient evaluations using growing batches (which improves performance in the early iterations) and support vectors (which improves performance in the later iterations). The third part of this thesis switches to the problem of hyper-parameter tuning. Although it is a lower dimensional problem, it can be more difficult as it is a non-convex global optimization problem. First, we propose a harmless global optimization algorithm to ensure that the performance is not worse than using random search. Moreover, we explore the use of gradient-based Bayesian optimization (BO) to improve the performance. We propose the use of directional derivatives to address the memory issues of BO. Finally, in the fourth part of this thesis, we propose a novel optimization method that combines the advantages of BO and Lipschitz optimization (LO).
View record
We study the problem of decision-making under uncertainty in the bandit setting. This thesis goes beyond the well-studied multi-armed bandit model to consider structured bandit settings and their applications. In particular, we learn to make better decisions by leveraging the application-specific problem-structure in the form of features or graph information. We investigate the use of structured bandits in two practical applications: online recommender systems with an available network of users and viral marketing in social networks. For each of these applications, we design efficient bandit algorithms and theoretically characterize their performance. We experimentally evaluate the efficiency and effectiveness of these algorithms on real-world datasets. For applications that require modelling complex non-linear feature-reward relationships, we propose a bootstrapping approach and establish theoretical regret bounds for it. Furthermore, we consider the application of multi-class classification with bandit feedback as a test-bed for evaluating our bootstrapping approach.
View record
This work looks at large-scale machine learning, with a particular focus on greedy methods. A recent trend caused by big datasets is to use optimization methods that have a cheap iteration cost. In this category are (block) coordinate descent and Kaczmarz methods, as the updates of these methods only rely on a reduced subspace of the problem at each iteration. Prior to our work, the literature cast greedy variations of these methods as computationally expensive with comparable convergence rates to randomized versions. In this dissertation, we show that greed is good. Specifically, we show that greedy coordinate descent and Kaczmarz methods have efficient implementations and can be faster than their randomized counterparts for certain common problem structures in machine learning. We show linear convergence for greedy (block) coordinate descent methods under a revived relaxation of strong convexity from 1963, which we call the Polyak-Lojasiewicz (PL) inequality. Of the proposed relaxations of strong convexity in the recent literature, we show that the PL inequality is the weakest condition that still ensures a global minimum. Further, we highlight the exploitable flexibility in block coordinate descent methods, not only in the different types of selection rules possible, but also in the types of updates we can use. We show that using second-order or exact updates with greedy block coordinate descent methods can lead to superlinear or finite convergence (respectively) for popular machine learning problems. Finally, we introduce the notion of “active-set complexity”, which we define as the number of iterations required before an algorithm is guaranteed to reach the optimal active manifold, and show explicit bounds for two common problem instances when using the proximal gradient or the proximal coordinate descent method.
View record
Theses completed in 2010 or later are listed below. Please note that there is a 6-12 month delay to add the latest theses.
Optimization of deep neural networks has been studied extensively, but our understanding of it is still very limited. In particular, it is still unclear how to optimally set hyperparameters such as the step size for gradient descent when training neural networks. We explore the issue of tuning the step size for full batch gradient descent, examining a proposed phenomenon in the literature called the "edge of stability". This refers to a phase of training for neural networks when the training loss non-monotonically decreases over time while the sharpness (the maximum eigenvalue of the Hessian matrix) oscillates around the threshold of precisely two divided by the step size. In this thesis, we start by providing the necessary background to understand the current state of the art in this area. We then perform various experiments on the sharpness, and study its trajectory over the course of training with full batch gradient descent. Unlike the vast majority of the previous literature, we focus on investigating the sharpness with respect to the various layers of neural networks individually. We observe that different layers of a neural network have differing behavior in their sharpness trajectories. We focus on fully connected networks and examine how varying hyperparameters of the network, such as the number of layers or hidden units per layer, impact the sharpness. Finally, we explore how various architecture choices impact step size selection when training with gradient descent. We see that changing just one parameter of a deep neural network, such as the non-linear activation functions used in each layer, can greatly impact which step sizes can lead to divergence during training. This motivates further investigation into how architecture choices affect hyperparameter selection.
View record
Reinforcement learning (RL) is an area of machine learning where intelligent agents take sequential actions in an environment and learn from experience to maximize rewards. This is particularly useful when the dynamics of the environment are complex and explicit training data is limited, such as in autonomous driving, robotics, game-playing, recommendation systems, finance and health care. The main challenge in RL is to balance between exploration (taking less observed actions to gather information) and exploitation (taking actions with high historical reward). Stochastic Multi-arm bandits (MABs) can be viewed as a simplified decision-making problem where the state of the agent does not change by the actions. The rich existing bandit theory provides insights into tackling expiration-exploitation trade-offs in RL.In this work, we draw inspiration from the classical bandit algorithms upper confidence bound (UCB)and Thompson sampling (TS), and propose a novel algorithm in RL: Optimistic Thompson sampling. Optimism encourages exploration: Agents can gain information by being optimistic and playing actions that are estimated to be sub-optimal but are not sufficiently sampled. We first discuss our performance metric in theoretical analysis, namely regret. Regret measures how the algorithm performs compared to the optimal strategy if the rewards and dynamics of the environment are known. We then provide a novel theoretical analysis of the optimistic Thompson sampling (O-TS) [Chapelle and Li, 2011] algorithms in bandits. Next, we extend the algorithms to the Markov decision process (MDP) setting, a common representation for RL problems. We propose two novel algorithms, O-TS-MDP and O-TS-MDP+. We compare their performance to existing work both theoritically and with numerical experiments. Finally, we conclude our work with a discussion of future directions for strategic exploration in bandits and RL.
View record
Many machine learning (ML) problems are solved by iteratively optimizing an objective along a single descent direction. By restricting the search for the next iterate to one direction, per iteration cost is kept low. In many important problem classes, however, it is possible to perform subspace optimization (SO) and search along additional directions nearly "for free". This can lead to finding a better next iterate and result in fewer iterations to reach a solution. In theory and in practice, however, SO is rarely used and existing theory usually assumes an exact SO. In this thesis, we show experimentally that SO could lead to more accurate solutions in less time. We also explore the effect of different SO parameter settings. The experiments are performed through a software package we developed called minFuncLinear which is available for download. On the theory side, we propose a more abstract problem class, multilinear map problems, where SO could be performed efficiently. This encompasses linear composition models, known to allow efficient SO. We give further examples of problems that fall under this framework, such as log-determinant problems. We also provide preliminary convergence analysis of gradient descent (GD) combined with SO, discuss multi-dimensional Wolfe conditions and extend initialization and interpolation methods from linesearches to subspace searches.
View record
Current machine learning practice requires solving huge-scale empirical risk minimization problems quickly and robustly. These problems are often highly under-determined and admit multiple solutions which exactly fit, or interpolate, the training data. In such cases, stochastic gradient descent has been shown to converge without decreasing step-sizes or averaging, and can achieve the fast convergence of deterministic gradient methods. Recent work has further shown that stochastic acceleration and line-search methods for step-size selection are possible in this restricted setting. Although pioneering, existing analyses for first-order methods under interpolation have two major weaknesses: they are restricted to the finite-sum setting, and, secondly, they are not tight with the best deterministic rates. To address these issues, we extend the notion of interpolation to stochastic optimization problems with general, first-order oracles. We use the proposed framework to analyze stochastic gradient descent with a fixed step-size and with an Armijo-type line-search, as well as Nesterov’s accelerated gradient method with stochastic gradients. In nearly all settings, we obtain faster convergence with a wider range of parameters. The improvement for stochastic Nesterov acceleration is comparable to dividing by the square-root of the condition number and addresses criticism that existing rates were not truly “accelerated”. In the case of convex functions, our convergence rates for stochastic gradient descent — both with and without the stochastic Armijo line-search — recover the best-known rates in the deterministic setting. We also provide a simple extension to L2-regularized minimization, which opens the path to proximal-gradient methods and non-smooth optimization under interpolation.
View record
Natural language processing (NLP) has pervasive applications in everyday life, and has recently witnessed rapid progress. Incorporating latent variables in NLP systems can allow for explicit representations of certain types of information. In neural machine translation systems, for example, latent variables have the potential of enhancing semantic representations. This could help improve general translation quality. Previous work has focused on using variational inference with diagonal covariance Gaussian distributions, which we hypothesize cannot sufficiently encode latent factors of language which could exhibit multi-modal distributive behavior. Normalizing flows are an approach that enables more flexible posterior distribution estimates by introducing a change of variables with invertible functions. They have previously been successfully used in computer vision to enable more flexible posterior distributions of image data. In this work, we investigate the impact of normalizing flows in autoregressive neural machine translation systems. We do so in the context of two currently successful approaches, attention mechanisms, and language models. Our results suggest that normalizing flows can improve translation quality in some scenarios, and require certain modelling assumptions to achieve such improvements.
View record
We consider stochastic second-order methods for minimizing smooth and strongly-convex functions under an interpolation condition, which can be satisfied by over-parameterized machine learning models. Under this condition, we show that the regularized subsampled Newton’s method (R-SSN) achieves global linear convergence with an adaptive step-size and a constant batch-size. By growing the batch size for both the subsampled gradient and Hessian, we show that R-SSN can converge at a quadratic rate in a local neighbourhood of the solution. We also show that R-SSN attains local linear convergence for the family of self-concordant functions. Furthermore, we analyze stochastic BFGS algorithms in the interpolation setting and prove their global linear convergence. We empirically evaluate stochastic limited-memory BFGS (L-BFGS) and a “Hessian-free” implementation of R-SSN for binary classification on synthetic, linearly-separable datasets and real datasets under a kernel mapping. Our experimental results demonstrate the fast convergence of these methods, both in terms of the number of iterations and wall-clock time.
View record
The generation of data has traditionally been specified using hand-craftedalgorithms. However, oftentimes the exact generative process is unknownwhile only a limited number of samples are observed. One such case isgenerating images that look visually similar to an exemplar image or as ifcoming from a distribution of images. We look into learning the generatingprocess by constructing a similarity function that measures how close thegenerated image is to the target image. We discuss a framework in whichthe similarity function is specified by a pre-trained neural network withoutfine-tuning, as is the case for neural texture synthesis, and a frameworkwhere the similarity function is learned along with the generative processin an adversarial setting, as is the case for generative adversarial networks.The main point of discussion is the combined use of neural networks andmaximum mean discrepancy as a versatile similarity function. Additionally, we describe an improvement to state-of-the-art style transferthat allows faster computations while maintaining generality of the generatingprocess. The proposed objective has desirable properties such as a simpleroptimization landscape, intuitive parameter tuning, and consistent frame-by-frame performance on video. We use 80,000 natural images and 80,000paintings to train a procedure for artistic style transfer that is efficient butalso allows arbitrary content and style images.
View record
If this is your researcher profile you can log in to the Faculty & Staff portal to update your details and provide recruitment preferences.