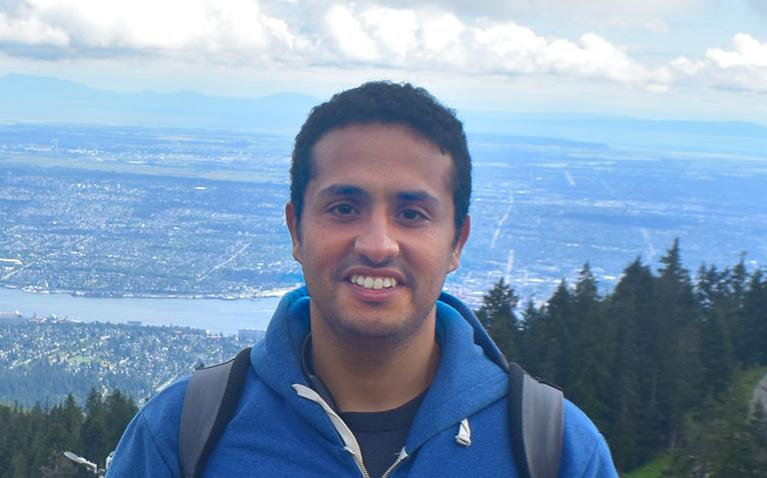
Khaled Ahmed
Doctor of Philosophy in Electrical and Computer Engineering (PhD)
Research Topic
Protecting Mobile Phone Users Against Malware
Dissertations completed in 2010 or later are listed below. Please note that there is a 6-12 month delay to add the latest dissertations.
The past decade has seen tremendous growth in how much data is collected and stored, and consequently in the sizes of application working sets. On-chip memory capacities, however, have not kept up: average CPU last-level cache capacity per core (thread) has stagnated at 1MB. Similar trends exist in special-purpose computing systems, with only up to tens of megabytes of on-chip memory available in most recent AI accelerators.In this dissertation, we explore hardware-friendly online data compression techniques to gain the performance benefits of larger on-chip memories without paying the costs of larger silicon. We propose several solutions including two methods to compress general workloads in CPU caches, and two methods to compress AI workloads in special-purpose computing systems. In order to efficiently compress on-chip data, the compression mechanisms need to leverage all relevant data stored in on-chip memory. We propose 2DCC, a cache compression mechanism that leverages redundancy both within and across all cache data blocks, and results in a 2.12× compression factor. We then extend this insight by observing that many on-chip blocks are often similar to each other. We propose Thesaurus, a hardware- level on-line cacheline clustering mechanism to dynamically form clusters as these similar blocks appear in the data access stream. Thesaurus significantly improves the state-of-the-art cache compression ratio to 2.25×. Next, we apply our insights to special-purpose applications. We first pro- pose Channeleon, which tackles the problem of compressing the activation maps in deep neural networks (DNNs) at inference time. Leveraging the observed similarity among activation channels, Channeleon first forms clusters of similar activation channels, and then quantizes activations within each cluster. This enables the activations to have low bit-width while incurring acceptable accuracy losses. Lastly, we propose Procrustes, a sparse DNN training accelerator that prunes weights by exploiting both software and hardware knowledge. Procrustes reduces the memory footprint of models by an order of magnitude while maintaining dense model accuracy.
View record
The Graphics Processing Unit (GPU) has become a mainstream computing platform for a wide range of applications. Unlike latency-critical Central Processing Units (CPUs), throughput-oriented GPUs provide high performance by exploiting massive application parallelism. In parallel programming, synchronization is necessary to exchange information for inter-thread dependency. However, inefficient synchronization support can serialize thread execution and restrict parallelism significantly. Considering parallelism is key to GPU performance, we aim to provide efficient and reliable synchronization support for both single-GPU and multi-GPU systems. To achieve this target, this dissertation explores multiple abstraction layers of computer systems, including programming models, memory consistency models, cache coherence protocols, and application specific knowledges of graphics rendering.First, to reduce programming burden without introducing data-races, we propose Relativistic Cache Coherence (RCC) to enforce Sequential Consistency (SC). By avoiding stalls of write permission acquisition with logical timestamps, RCC is 30% faster than the best prior SC proposal, and only 7% slower than the best non-SC design. Second, we introduce GETM, the first GPU Hardware Transactional Memory (HTM) with eager conflict detection, to help programmers implement deadlock-free, yet aggressively parallel code. Compared to the best prior GPU HTM, GETM is up to 2.1× (1.2× gmean) faster, area overheads are 3.6× lower, and power overheads are 2.2× lower. Third, we design HMG, a hierarchical cache coherence protocol for multi-GPU systems. By leveraging the latest scoped memory model, HMG not only can avoid full cache invalidation of software coherence protocol, but also filters out write invalidation acknowledgments and transient coherence states. Despite minimal hardware overhead, HMG can achieve 97% of the performance of an idealized caching system. Finally, we propose CHOPIN, a novel Split Frame Rendering (SFR) scheme by taking advantage of the parallelism of image composition. CHOPIN can eliminate the performance overheads of primitive duplication and sequential primitive distribution that exist in previous work. CHOPIN outperforms the best prior SFR implementation by up to 56% (25% gmean) in an 8-GPU system.
View record
Theses completed in 2010 or later are listed below. Please note that there is a 6-12 month delay to add the latest theses.
Today, nearly all modern devices, including smartphones, PCs, and cloud servers, benefit significantly from two architectural advancements that speed up computation. First, speculative execution allows the execution of instructions ahead of time; and second, cache memory store data close to the processor to reduce latency of memory operations. Unfortunately, these features also make compute systems vulnerable to security holes known as transient side-channel attacks. These attacks exploit predictive mechanisms and use mis-speculated instructions to modify the cache persistently as a side channel. Such attacks enables leakage of private data, such as cryptographic keys.Full-fledged mitigations against transient attacks have come at a significant performance cost. They either try to stop potential leakage at the source — where it is speculatively execution instructions — and penalize many “innocent” executions or laboriously restore past states in the memory hierarchy.This research focuses on mitigating the transient side-channel attacks while minimizing performance loss. Our approach combines significantly more efficient protection schemes inside the cache hardware, the destination, to stop potential leaks. We identify and leverage a specific memory access pattern to detect an ongoing cache side-channel attack and localize the protection to where it occurs.We propose FantôMiss, our mitigation strategy that allows cache state changes speculatively but also tracks the modified cache lines under speculation. Then, for subsequent accesses to those speculated cache lines, we generate fake cache misses that traverse the memory hierarchy that conceals speculative cache states, thereby closing the side-channel.Crucially, the increased latency is not imposed upon all load instructions but only on loads that read potential leak sources. As a result, FantôMiss significantly outperforms prior proposed mitigations, with execution-time overheads of just 0.5% and 1.6%(geometric-mean) across the PARSEC and SPEC benchmark suites.
View record
The emergence of deep learning has launched many works in deep learning accelerators. To fully realize the potential of these accelerators, dataflow mapping must be optimized in order to reduce the number of memory accesses. Dataflow mapping is crucial to improving the performance of deep learning workloads, but mapping optimization is a difficult problem due to the enormous, non-convex, and non-differentiable search space. As workloads become larger and larger, the problem becomes harder while the importance of dataflow increases.To tackle the problem, prior work reduces the search space using empirically driven, or arbitrary heuristics. However, these heuristics are either too simple and the optimization process is still too slow, or are too aggressive and remove optimal mappings. Prior work also explored using black-box optimizers, but reformulating the problem into the input of these black-box optimizers is not always feasible and scalable, leading to sub-optimal or even invalid solutions.In this thesis, we tackle the problem by first formally analyzing how the different aspects of mapping (tiling, ordering, unrolling) algebraically affect memory reuse and performance in order to identify sub-optimal spaces. Next, we introduce new state-space representations and traversal methods to enable the pruning of these spaces, which dramatically reduces the search space without rejecting the best solutions. Finally, we extend these analyses and techniques to tackle problems closely related to mapping optimization, such as memory configuration optimization.Sunstone, our proof-of-concept implementation, improves the optimization time for some of the complex tensor operations by up to 10x compared to prior work, and can yield mappings with up to 1.5-2.5x lower EDP.
View record
Improving the spectral efficiency is a key challenge to meet the increasing demandfor higher capacity over communication channels. Faster than Nyquist (FTN) signaling(first proposed in 1970s) has recently regained popularity due to its abilityto improve signal’s spectral efficiency. FTN can achieve a symbol rate faster thanNyquist rate; therefore, it has been widely investigated in high-capacity wirelessand optical communications.FTN signaling comes at the cost of intersymbol interference (ISI), which requirescomplex decoder at the receiver to recover the received symbol from ISInoise. This decoder is a bottleneck of the system throughput since it is on the criticalpath of receiving a noise free signal. Several decoders have been proposed inthe literature to mitigate the ISI noise, however, these systems require a significantcomputational complexity to equalize the received frames.In this thesis, we propose a hardware architecture that implement an FTN decodersystem. This system consists of a Maximum A-posteriori (MAP) equalizerand low-density parity (LDPC) decoder that work together iteratively to mitigateISI noise and channel noise. The MAP equalizer is mainly responsible for equalizingISI noise, while the LDPC decoder uses parity checks to remove randomchannel noise.We study the design trade-offs for each block, evaluate it separately, and proposea high-throughput hardware architecture for the FTN decoder system. Weevaluate this architecture on Xilinx UltraScale+ (xcvu13p) device. Our MAP equalizerachieves a throughput up to 602 Mbps per processing element (PE). We alsodesign an LDPC decoder that achieves a throughput of up to 520 Mbps per PE. TheFTN decoder architecture achieves a throughput of up to 2.16 Gbps.
View record
Caches are essential to today's microprocessors. They close the huge speed gap between processors and memories. However, cache design presents an important tradeoff. A bigger cache size should increase performance and allow processors to perform faster, but it is also limited by its silicon, area, and power consumption costs. Today's caches often use half of the silicon area in processor chips and consume a lot of power. Instead of physically increasing the cache size, effective cache capacity can be substantially increased if the data inside the cache is compressed.Current cache compression techniques focus only on one granularity, either compressing inside one cache line, or compressing similar cache lines together. In this work, we combine both compression techniques to leverage both inter-line and intra-line compression. We find that combining both techniques results in better compression than previously described methods, and also maintains the same performance as a normal uncompressed cache when running incompressible applications. We study and address the design considerations and tradeoffs that arise from such design. We address issues related to the design like cache structure and replacement policies. Then we present an implementation that achieves the best possible compression and performance while maintaining overheads as low as possible.
View record
In recent years, neural networks have regained popularity in a variety of fields such as image recognition and speech transcription. As deep neural networks grow more popular for solving everyday tasks, deployment on small embedded devices — such as phones — is becoming increasingly popular. Moreover, many applications — such as face recognition or health applications — require personalization, which means that networks must be retrained after they have been deployed.Because today’s state-of-the-art networks are too large to fit on mobile devices and exceed mobile device power envelopes, techniques such as pruning and quantization have been developed to allow pre-trained networks to be shrunk by about an order of magnitude. However, they all assume that the network is first fully trained off-line on datacenter-class GPUs, then pruned in a post-processing step, and only then deployed to the mobile device.In this thesis, we introduce DropBack, a technique that significantly reduces the storage and computation required during both inference and training. In contrast to existing pruning schemes, which retain the weights with the largest values and set the rest to zero, DropBack identifies the weights that have changed the most, and recomputes the original initialization values for all other weights. This means that only the most important weights must be stored in off-chip memory both during inference and training, reducing off-chip memory accesses (responsible for a majority of the power usage) by up to 72×.Crucially, networks pruned using DropBack maintain high accuracy even for challenging network architectures: indeed, on modern, compact network architectures such as Densenet and WRN-28-10, DropBack outperforms the current state-of-the- art pruning techniques in both accuracy and off-chip memory storage required for weights. On the CIFAR-10 dataset, we observe 5× reduction in weights on an already 9×-reduced VGG-16 network, which we call VGG-S, and 4.5× on Densenet and WRN-28-10 — all with zero or negligible accuracy loss — or 19×, 27×, and 36×, respectively, with a minor impact on accuracy. When the recomputed initial weights are decayed to zero, the weight memory footprint of WRN-28-10 can be reduced up to 72×.
View record
If this is your researcher profile you can log in to the Faculty & Staff portal to update your details and provide recruitment preferences.