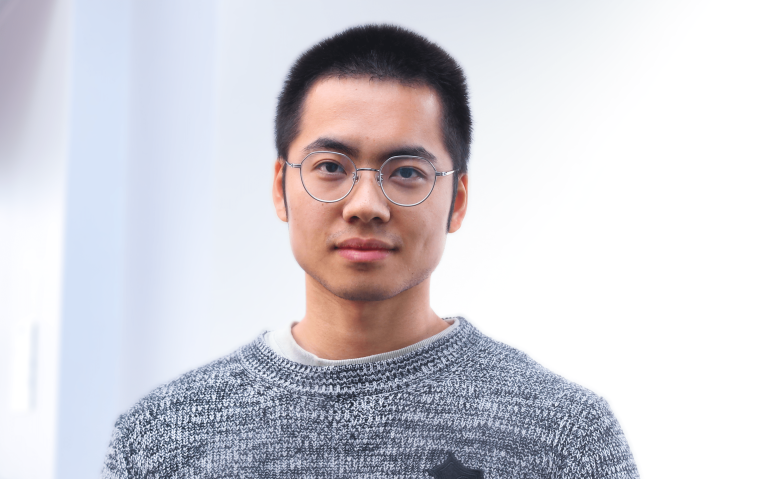
Zitao Chen
Doctor of Philosophy in Electrical and Computer Engineering (PhD)
Research Topic
Making machine learning reliable, secure and private
Dissertations completed in 2010 or later are listed below. Please note that there is a 6-12 month delay to add the latest dissertations.
Despite the prominence of automated runtime configuration procedures, relatively little is known about managing the runtime configurations of general-purpose programming in resource-constrained IoT platforms at the network edge. For example, high-level language-written application programming (e.g., video/audio surveillance) in IoT enables local data processing to decrease latency, bandwidth, and infrastructure costs and address data safety and privacy concerns. However, without a good configuration, such computing generates undesirable performance or sudden and unexpected resource outages, leading to an application or a complete system failure. On the other hand, stringent resources in IoT make the performance of general-purpose programming highly discontinuous, which the existing linear or non-linear models can not capture. As a result, while the current configuration techniques make typical computing (e.g., cloud, High-Performance Computing (HPC)) efficient, it still needs to be determined whether or not they are efficient enough to manage general-purpose edge computing.This research systematically analyzed the runtime configuration challenges for general-purpose programming in IoT. In the process, we discovered several new application performance associations and system resource variance patterns in this state space with which we address the constraints, heterogeneity, discontinuity, and scalability issues of IoT at the network edge. We applied these performance associations and other systematic state space sampling methods to address these issues as they arise in two important and prominent areas of automated runtime configuration: (1) resource-exhaustion detection and (2) performance optimization. The latter area is divided more into a pipeline configuration and b collocated performance approximation.With cross-platform failure prediction, configuration management, and approximation techniques, we apply an intelligent and general set of configuration capabilities to general-purpose edge computing. Across various real-world case studies, our techniques outperform conventional runtime configuration techniques regarding performance improvements and approximation accuracy and pave the way for a new direction toward general-purpose edge computing.
View record
The growth in the popularity of smart contracts has been accompanied by a rise in security attacks targeting vulnerabilities in smart contracts, which led to financial losses of millions of dollars and erosion of trust. To enable developers find vulnerabilities in the code of smart contracts, researchers and industry practitioners have proposed several static analysis tools. However, vulnerabilities abound in smart contracts, and the effectiveness of the state-of-the-art analysis tools in detecting vulnerabilities has not been studied.To understand the effectiveness of the state-of-the-art static analysis tools in detecting vulnerabilities in smart contracts, we propose a systematic approach for evaluating smart contract static analysis tools using security bug injection. We use our proposed approach to evaluate the effectiveness of well-known static analysis tools. The evaluation results show that analysis tools fail to detect significant vulnerabilities and report a high number of false alarms. To improve the state of static analysis for finding vulnerabilities, we expand the space of vulnerability detection and propose static analysis approaches for detecting two-broad categories of vulnerabilities in smart contracts, namely, gas-related vulnerabilities and access control vulnerabilities. Our proposed solutions rely on identifying security properties in the code of smart contracts and then analyzing the dependency of the contract code on user inputs that lead to violating the identified security properties. The results show that our proposed vulnerability detection approaches achieve a significant improvement in the effectiveness of detecting vulnerabilities compared to the prior work.
View record
There now exists more than eight billion IoT devices with expected growth to reach over 22 billion by 2025. IoT devices are comprised of sensor and actuator components which generate live-stream data and share information via a common communication link, e.g., the Internet. For example, in a smart home, a number of IoT devices such as a Google Home/Amazon Alexa, smart plugs, security cameras, a garage door, and a thermostat connect to the WiFi network to routinely communicate with each other, share information, and take actions accordingly. However, a main security challenge is protecting shared information between authorized devices/users while distinguishing real objects from fake ones in the network. Such a challenge aggravates man-in-the-middle, and denial-of-service vulnerabilities. To defend such concerns, in this thesis, we first propose an authorization framework called Dynamic Policy-based Access Control (DynPolAC) as a model for protecting information in dynamic and resource-constrained IoT systems. We focus our experiments with DynPolAC on an IoT environment comprised of drones. DynPolAC achieves more than 7x speed performance improvements in authorization when compared to previously proposed methods for resource-constrained IoT platforms such as drones. Secondly, in this thesis, we implement a method called Phoenix to detect fake drones in an IoT network from real drones. We experimentally train and derive Phoenix from a control function called the Lyapunov stability function. We evaluate Phoenix for drones using an autopilot simulator as well as flying a real drone. We find that Phoenix takes about 50 ms to distinguish real drones from fake ones, while by asymmetry, it could take days for motivated attackersto reconstruct Phoenix. Phoenix also achieves a precision rate of 99.55% to detect real drones and a recall rate of 99.84% to detect fake drones.
View record
Transient hardware faults have become one of the major concerns affecting the reliability of modern high-performance computing (HPC) systems. They can cause failure outcomes for applications, such as crashes and silent data corruptions (SDCs) (i.e. the application produces an incorrect output). To mitigate the impact of these failures, HPC applications need to adopt fault tolerance techniques.The most common practices of fault tolerance techniques include (i) characterization techniques, such as fault injection and architectural vulnerability factor (AVF)/program vulnerability factor (PVF) analysis; (ii) run-time error detection techniques; and (iii) error recovery techniques. However, these approaches have the following shortcomings: (i) fault injections are generally time-consuming andlack predictive power, while the AVF/PVF analysis offers low accuracy; (ii) prior techniques often do not fully exploit the program’s error resilience characteristics; and (iii) the application constantly pays a performance/storage overhead.This dissertation proposes comprehensive approaches to improve the above techniques in terms of effectiveness and efficiency. In particular, this dissertation makes the following contributions: First, it proposes ePVF, a methodology that distinguishes crash-causing bits from the architecturally correct execution (ACE) bits and obtains a closer estimate of the SDC rate than PVF analysis (by 45% to 67%). To reduce the overall analysis time, it samples representative patterns from ACE bits and obtains a good approximation (less than 1% error) for the overall prediction. This dissertation applies the ePVF methodology to error detection, which leads to a 30% lower SDC rate than well-accepted hot-path instruction duplication.Second, this dissertation combines the roll-forward recovery and the roll-back recovery schemes and demonstrates the improvement in the overall efficiency of the C/R with two systems: LetGo (for faults affecting computational components) and BonVoision (for faults affecting DRAM memory). Overall, LetGo is able to elide 62% of the crashes caused by computational faults and convert them to continued execution (out of these 80% result in correct output while a majority of the rest fall back on the traditional roll-back recovery technique). BonVoision is able to continue to completion 30% of the DRAM memory detectable but uncorrectable errors (DUEs).
View record
Hardware errors are projected to increase in modern computer systems due to shrinking feature sizes and increasing manufacturing variations. The impact of hardware faults on programs can be catastrophic, and can lead to substantial financial and societal consequences. Error propagation is often the leading cause of catastrophic system failures, and hence must be mitigated. Traditional hardware only techniques to avoid error propagation are energy hungry, and hence not suitablefor modern computer systems (i.e., commodity systems). Researchers have proposed selective software-based protection techniques to prevent error propagation at lower costs. However, these techniques use expensive fault injection simulations to determine which parts of a program must be protected. Fault injection simulation artificially introduces a fault to program execution and observefailures (if any) upon the completion of the program execution. Thousands of such simulations need to be performed in order to achieve statistical significance. It is time-consuming as even a single program execution of a common application may take a long time. In this dissertation, I first characterize error propagation in programs that lead to different types of failures, proposed both empirical and analytical approaches to identify and mitigate error propagation without expensive fault injections. The key observation is that only a small fraction of states are responsible for almost all error propagation in programs, and the propagation falls into identifiable patterns which can be modeled efficiently. The proposed techniques are nearly as close as fault injection approaches in measuring failure rates of programs, and orders of magnitude faster than fault injections. This allows developers to build low-cost fault-tolerant applications in an extremely efficient manner.
View record
Program comprehension is crucial in software engineering; a necessary step for performing many tasks. However, the implicit and intricate relations between program entities hinder comprehension of program behaviour and change. It is particularly a difficult endeavour to understand dynamic and modern programming languages such as JavaScript, which has grown to be among the most popular languages. Comprehending such applications is challenging due to the temporal and implicit relations of asynchronous, DOM-related and event-driven entities spread over the client and server sides.The goal of the work presented in this dissertation is to facilitate program comprehension through the following techniques. First, we propose a generic technique for capturing low-level event-based interactions in a web application and mapping those to a higher-level behavioural model. This model is then transformed into an interactive visualization, representing episodes of execution through different semantic levels of granularity. Then, we present a DOM-sensitive hybrid change impact analysis technique for JavaScript through a combination of static and dynamic analysis. Our approach incorporates a novel ranking algorithm for indicating the importance of each entity in the impact set. Next, we introduce a method for capturing a behavioural model of full-stack JavaScript applications’ execution. The model is temporal and context-sensitive to accommodate asynchronous events, as well as the scheduling and execution of lifelines of callbacks. We present a visualization of the model to facilitate program comprehension for developers. Finally, we propose an approach for facilitating comprehension by creating an abstract model of software behaviour. The model encompasses hierarchies of recurring and application-specific motifs. The motifs are abstract patterns extracted from traces through our novel technique, inspired by bioinformatics algorithms. The motifs provide an overview of the behaviour at a high level, while encapsulating semantically related sequences in execution. We design a visualization that allows developers to observe and interact with inferred motifs.We implement our techniques in open-source tools and evaluate them through a set of controlled experiments. The results show that our techniques significantly improve developers’ performance in comprehending the behaviour and impact of change in software systems.
View record
Embedded systems are widely used in critical situations and hence, are targetsfor malicious users. Researchers have demonstrated successful attacksagainst embedded systems used in power grids, modern cars, and medicaldevices. Hence, it is imperative to develop techniques to improve securityof these devices. However, embedded devices have constraints (such as limitedmemory capacity) that make building security mechanisms for themchallenging.In this thesis, we formulate building Intrusion Detection System (IDS)for embedded systems as an optimization problem. We develop algorithmsthat, given the set of the security properties of the system and the invariantsthat verify those properties, build an IDS that maximizes the coverage forthe security properties, with respect to the available memory. This allowsour IDS to be applicable to a wide range of embedded devices with di erentmemory capacities. Furthermore, we develop techniques to analyze securityof both design and implementation of embedded systems. Given a set ofcapabilities of attackers, we automatically analyze the system and identifyways an adversary may tamper with the system. This will help developersdiscover new attacks, and improve the design and implementation of thesystem.
View record
With web application usage becoming ubiquitous, there is greater demand for making such applications more reliable. This is especially true as more users rely on web applications to conduct day-to-day tasks, and more companies rely on these applications to drive their business. Since the advent of Web 2.0, developers often implement much of the web application’s functionality at the client-side, using client-side JavaScript. Unfortunately, despite repeated complaints from developers about confusing aspects of the JavaScript language, little work has been done analyzing the language’s reliability characteristics. With this problem in mind, we conducted an empirical study of real-world JavaScript bugs, with the goal of understanding their root cause and impact. We found that most of these bugs are DOM-related, which means they occur as a result of the JavaScript code’s interaction with the Document Object Model (DOM). Having gained a thorough understanding of JavaScript bugs, we designed techniques for automatically detecting, localizing and repairing these bugs. Our localization and repair techniques are implemented as the AutoFLox and Vejovis tools, respectively, and they target bugs that are DOM-related. In addition, our detection techniques – Aurebesh and Holocron – attempt to find inconsistencies that occur in web applications written using JavaScript Model-View-Controller (MVC) frameworks. Based on our experimental evaluations, we found that these tools are highly accurate, and are capable of finding and fixing bugs in real-world web applications.
View record
Over three decades of continuous scaling in CMOS technology has led to tremendous improvements in processor performance. At the same time, the scaling has led to an increase in the frequency of hardware errors due to high process variations, extreme operating conditions and manufacturing defects. Recent studies have found that 40% of the processor failures in real-world machines are due to intermittent hardware errors. Intermittent hardware errors are non-deterministic bursts of errors that occur in the same physical location. Intermittent errors have characteristics that are different from transient and permanent errors, which makes it challenging to devise efficient fault tolerance techniques for them.In this dissertation, we characterize the impact of intermittent hardware faults on programs using fault injection experiments at the micro-architecture level. We find that intermittent errors are likely to generate software visible effects when they occur. Based on our characterization results, we build intermittent error tolerance techniques with focus on error diagnosis and recovery. We first evaluate the impact of different intermittent error recovery scenarios on a processor's performance and availability. We then propose DIEBA (Diagnose Intermittent hardware Errors in microprocessors by Backtracing Application), a software-based technique to diagnose the fault-prone functional units in a processor.
View record
Theses completed in 2010 or later are listed below. Please note that there is a 6-12 month delay to add the latest theses.
Heterogeneous edge platforms enable the efficient execution of machine learning inference applications. These applications often have a critical constraint (such as meeting a deadline) and an optimization goal (such as minimizing energy consumption). To navigate this space, existing optimization frameworks adjust the platform's frequency configuration for the CPU, the GPU and/or the memory controller. These optimization frameworks, however, are thermal-oblivious disregarding the fact that edge platforms are frequently deployed in environments where they are exposed to ambient temperature variations. In this thesis, we first characterize the impact of ambient temperature on the power consumption and execution time of machine learning inference applications running on a popular edge platform, the NVIDIA Jetson TX2. Our rigorous data collection and statistical methodology reveals a sizeable ambient temperature impact on power consumption (about 20\% on average, and up to 40\% on some workloads) and a moderate impact on runtime (up to 5\%). We also find that existing, thermal-oblivious optimization frameworks select frequency configurations that either violate the application's constraints and/or are sub-optimal in terms of the optimization goal assigned. To address these shortcomings, we propose EdgeEngine, a lightweight thermal-aware optimization framework. EdgeEngine monitors the platform's temperature and uses reinforcement learning to adjust the frequency configuration of all underlying platform resources to meet the application's constraints. We find that EdgeEngine meets the application's constraint, and achieves up to 29\% lower energy consumption (up to 2x) and up to 41\% fewer violations compared to existing state-of-the-art thermal-oblivious optimization frameworks.
View record
Swarm robotics, particularly drone swarms, are used in various safety-critical tasks (e.g., package delivery, warehouse management, entertainment, agriculture, etc). Drone swarms use swarm intelligence to carry out the mission in collaboration. While a lot of attention has been given to improving swarm control algorithms for improved intelligence, the security implications of various design choices in swarm control algorithms have not been studied. We highlight how an attacker can exploit the vulnerabilities in swarm control algorithms to disrupt drone swarms. GPS attack is a kind of physical attack that feeds the drone with erroneous GPS signals via physical channels such as the GPS signal emitter. Specifically, we show that the attacker can target a swarm member (target drone) through GPS spoofing attacks, and indirectly cause other swarm members (victim drones) to veer from their course, resulting in a collision. We call these Swarm Propagation Vulnerabilities.In this thesis, we introduce a fuzzing framework consisting of two fuzzing tools, SwarmFuzzGraph and SwarmFuzzBinary , to capture the attacker’s ability, and efficiently find such vulnerabilities in swarm control algorithms. SwarmFuzzGraph uses a combination of graph theory and gradient-guided optimization to find the potential attack parameters. Our evaluation on a popular swarm control algorithm shows that SwarmFuzzGraph achieves an average success rate of 48.8% in finding SPVs. Compared to random fuzzing, SwarmFuzzGraph has a 10x higher success rate, and 3x lower runtime. However, SwarmFuzzGraph fails to find SPVs when applied in another swarm control algorithm due to its inability to handle different swarm topologies. We then propose SwarmFuzzBinary , which uses observation-based seed scheduling and binary search to find attack parameters in swarms with various topologies. The evaluation on the same two swarm control algorithms shows that SwarmFuzzBinary ’s success rate is comparable to SwarmFuzzGraph and works in all tested algorithms. Specifically, SwarmFuzzBinary achieves an average success rate of 60.2% and 86.5% in each swarm control algorithm. Compared to random fuzzing, SwarmFuzzBinary has a 5x higher success rate, and 3x lower runtime. We also find that swarms of a larger size are more vulnerable to this attack type, for a given spoofing distance.
View record
Machine learning (ML) applications have been ubiquitouslydeployed across critical domains such as autonomous vehicles(AVs), and medical diagnosis. Vision-based ML models like ResNet are used for object classification and lane detection, while Large Language Models (LLMs) like ChatGPT are used in cars to enable robust and flexible voice commands in AVs. The use of ML models in safety-critical scenarios requires reliable ML models.In the first part of this thesis, we primarily focus on understanding the resilience of ML models against transient hardware faults in CPUs. Towards this end, we present an LLVM IR-level FI tool, LLTFI, which we use to evaluate the effect of transient faults on Deep Neural Networks (DNNs) and LLMs. We found that LLTFI is more precise than TensorFI, an application-level FI tool proposed by prior work. Unlike LLTFI, TensorFI underestimates the resilience of DNNs by implicitly assuming that every injected fault corrupts the outputs of the intermediate layers of the DNN. Using LLTFI, we also evaluated the efficacy of Selective Instruction Duplication to make DNNs more resilient against transient faults. While in the case of DNNs, transient faults cause the model to misclassify or mispredict the object, for LLMs, we found transient faults to cause the model to produce semantically and syntactically incorrect outputs.In the second part of this thesis, we evaluate the effect of permanent stuck-at faults in systolic arrays on DNNs. We present a Register Transfer (RTL)-Level FI tool, called SystoliFI, to inject permanent stuck-at faults in the systolic array, which we use to understand the manifestation of stuck-at faults in systolic arrays in the intermediate layers of the DNNs. We found that the manifestation of the stuck-at faults varies significantly with the type of operation (Convolution vs. Matrix multiplication), the operation size, and the systolic array size.
View record
Convolutional Neural Networks (CNNs) are broadly used in safety-critical applications such as autonomous vehicles. While demonstrating high accuracy, CNN models are vulnerable to Dynamic Random Access Memory (DRAM) errors corrupting their parameters, thereby degrading their accuracy. Unfortunately, existing techniques for protecting CNNs from memory errors are either costly or not complete, meaning that they fail to protect from large-granularity, multi-bit DRAM errors.In this thesis, we propose a software-implemented coding scheme, Structural Coding, which is able to achieve three orders of magnitude reduction in Silent Data Corruption (SDC) rates of CNNs under large-granularity memory errors. Its error correction coverage is also significantly higher than other software-techniques to protect CNNs from faults in the memory. Additionally, its average performanceoverhead on a real machine is less than 3%. The memory footprint overhead of Structural Coding is
View record
The Ethereum blockchain stores and executes complex logic via smart contracts written in Solidity, a high-level programming language. The Solidity language provides features to exercise fine-grained control over smart contracts, termed low-level functions. However, the high-volume of transactions and the improper use of low-level functions lead to security exploits with heavy financial losses. Consequently, the Solidity community has suggested secure alternatives to low-level functions.In this thesis, we first perform an empirical study on the use of low-level functions in Ethereum smart contracts. We study a smart contract dataset consisting of over 2,100,000 real-world smart contracts. We find that low-level functions are widely used and that 95% of these uses are gratuitous, and are hence replaceable. We then propose GoHigh, a source-to-source transformation tool to eliminate low-level function-related vulnerabilities, by replacing low-level functions with secure high-level alternatives. Our experimental evaluation on the dataset shows that, among all the replaced contracts, about 80% of them do not introduce unintended side-effects, and the remaining 20% are not verifiable due to their external dependencies. Further, GoHigh saves more than 5% of the gas cost of the contract after replacement. Finally, GoHigh takes 7 seconds on average per contract.
View record
Graphics Processing Units (GPUs) are popular for reliability-conscious uses in High Performance Computing (HPC), machine learning algorithms, and safety-critical applications. Fault injection (FI) techniques are generally used to determine the reliability profiles of programs in the presence of soft errors. However, these techniques are highly resource- and time-intensive. GPU applications are highly multi-threaded and typically execute hundreds of thousands of threads, which makes it challenging to apply FI techniques. Prior research developed a model called TRIDENT to analytically predict Silent Data Corruption (SDC) (i.e., incorrect output without any indication) probabilities of single-threaded CPU applications, without requiring any FIs. Unfortunately, TRIDENT is incompatible with GPU programs, due to their high degree of parallelism and different memory architectures compared to CPU programs. The main challenge is that modeling error propagation across thousands of threads in a Graphics Processing Unit (GPU) kernel requires enormous amounts of data to be profiled and analyzed, posing a major scalability bottleneck for HPC applications. Further, there are GPU-specific behaviors that must be modeled for accuracy. In this thesis, we propose GPU-TRIDENT, an accurate and scalable technique for modeling error propagation in GPU programs. Our key insight is that error propagation across threads can be modeled based on program execution patterns. These can be characterized by control-flow, loop iteration, data, and thread block patterns of the GPU program. We also identify two major sources of inaccuracy in building analytical models of error propagation and mitigate them to improve accuracy. We find that GPU-TRIDENT can predict the SDC probabilities of both the overall GPU programs and individual instructions accurately, and is two orders of magnitude faster than FI-based approaches. We also demonstrate that GPUTRIDENT can guide selective instruction duplication to protect GPU programs similar to FI. We also deploy GPU-TRIDENT to assess the input-dependence of reliability of GPU kernels and find that the SDC probability of kernels is generally insensitive to variation in inputs.
View record
As Machine Learning (ML) has seen increasing adoption in safety-critical domains (e.g., autonomous vehicles), the reliability of ML systems has also grown in importance. While prior studies have proposed techniques to enable efficient error-resilience (e.g., selective instruction duplication), a fundamental requirement for realizing these techniques is a detailed understanding of the application's resilience.The primary part of this thesis focuses on studying ML application resilience to hardware and software faults. To this end, we present the TensorFI tool set, consisting of TensorFI 1 and 2 which are high-level fault injection frameworks for TensorFlow 1 and 2 respectively. With this tool set, we inject faults in TensorFlow programs and study important reliability aspects such as model resilience to different kinds of faults, operator and layer level resilience of different models or the effect of hyperparameter variations. We evaluate the resilience of 12 ML applications, including those used in the autonomous vehicle domain. From our experiments, we find that there are significant differences between different ML applications and different configurations. Further, we find that applications are more vulnerable to bit-flip faults than other kinds of faults. We conduct four case studies to demonstrate some use cases of the tool set. We find the most and least resilient image classes to faults in a traffic sign recognition model. We consider layer-wise resilience and observe that faults in the initial layers of an application result in higher vulnerability. In addition, we visualize the outputs from layer-wise injection in an image segmentation model, and are able to identify the layer in which faults occurred based on the faulty prediction masks. These case studies thus provide valuable insights into how to improve the resilience of ML applications.The secondary part of this thesis focuses on studying ML application resilience to data faults (e.g. adversarial inputs, labeling errors, common corruptions/noisy data). We present a data mutation tool, TensorFlow Data Mutator (TF-DM), which targets different kinds of data faults commonly occurring in ML applications. We conduct experiments using TF-DM and outline the resiliency analysis of different models and datasets.
View record
The past decade has witnessed the rise of the Internet of Things (IoT) devices with single-board computers such as the Raspberry Pi. The increased programmability and connectivity allow realization of the edge computing paradigm, in which we run on these devices complex distributed applications that were traditionally run on the cloud.Since IoT devices are subject to resource constraints like available battery power, we need to dynamically migrate a running process from one machine to another to prevent losing state. In cloud systems, VM migration techniques based on virtual memory snapshots are used in similar failure scenarios. However, it is challenging to apply virtualization-based migration techniques in the IoT domain, due to the differences in processor and platform architecture between IoT devices.In this thesis, we present a platform-independent migration technique, which we call ThingsMigrate, to address this challenge. Given a program, ThingsMigrate automatically instruments the source code to expose the hidden states such as closures and continuations. During run-time, the instrumented program produces on demand a platform-agnostic snapshot of the process, from which new code is generated to resume execution. Thus, ThingsMigrate enables process migration without any modifications to the underlying virtual machine, providing platform-independence. Using standard JavaScript (JS) benchmarks, we demonstrate that it can migrate resource-intensive applications, with average run-time latency overhead of 33% and memory overhead of 78%. ThingsMigrate supports multiple subsequent migrations without introducing additional overhead over each subsequent migration.
View record
Cyber-Physical Systems (CPS) are deployed in many mission-critical applications such as medical devices (e.g., an Artificial Pancreas System (APS)), autonomous vehicular systems (e.g., self-driving cars, unmanned aerial vehicles) and aircraft control management systems (e.g., Horizontal Collision Avoidance System (HCAS) and Collision Avoidance System-Xu (ACAS-XU)). Ensuring correctness is becoming more difficult as these systems adopt new technology, such as Deep Neural Network (DNN), to control these systems. DNN are black-box algorithms whose inner workings are complex and difficult to discern. As such, understanding their vulnerabilities is also complex and difficult.We identify a new vulnerability in these systems and demonstrate how to synthesize a new category of attacks Ripple False Data Injection Attacks (RFDIA) in them by perturbing specific inputs, by minimal amounts, to stealthily change the DNN’s output. These perturbations propagate as ripples through multiple DNN layers and can lead to corruptions that can be fatal. We demonstrate that it is possible to construct such attacks efficiently by identifying the DNN’s critical inputs. The critical inputs are those that affect the final outputs the most on being perturbed. Understanding this new class of attacks sets the stage for developing methods to mitigate vulnerabilities.Our attack synthesis technique is based on modeling the attack as an optimization problem using Mixed Integer Linear Programming (MILP). We define an abstraction for DNN-based CPS that allows us to automatically: 1) identify the critical inputs, and 2) find the smallest perturbations that produce output changes. We demonstrate our technique on three practical CPS with two mission-critical applications in increasing order of complexity: Medical systems (APS) and aircraft control management systems (HCAS and ACAS-XU). Our key observations for scaling our technique to complex systems such as ACAS-XU were to define: 1) appropriate intervals for their inputs and the outputs, and 2) attack specific objective (cost) functions in the abstraction.
View record
Robotic vehicles (RV) are increasing in adoption in many industrial sectors (e.g., agriculture, surveillance, package delivery, warehouse management, cinematography, etc). RVs use auto-pilot software for perception and navigation, and rely on sensors and actuators for operating autonomously in the physical world. As RVs rely on sensor measurements for actuation, a common way of triggering attacks against RVs is through sensor tampering or spoofing. Such attacks cannot be prevented through traditional software security methods (e.g., cryptography, memory isolation, etc). Real-time invariant analysis has been proved effective in detectingsensor tampering attacks against CPS such as smart meters, water treatment plants, and smart grids. Because RVs inherently use control algorithms for minimizing sensor or actuator faults and for trajectory planning, control-based invariant analysis techniques have been proposed to detect attacks against RVs. In this thesis, we evaluate the efficacy of control-based intrusion detection techniques, and propose three kinds of stealthy attacks that evade detection and disrupt RV missions. By design, control-based techniques perform threshold analysis to tolerate environmental noise e.g., wind, friction, etc. Our main insight is that due to model inaccuracies, control-based intrusion detection techniques have a high detection threshold to avoid false positives. We propose automated process by which an attacker can learn the thresholds, and consequently perform targeted attacks against the RV. We also present algorithms for performing the attacks without requiring the attacker to expend significant effort or know specific details of the RV, making the attacks applicable to a wide range of RVs. We demonstrate the attackson eight RV systems including three real vehicles, in the presence of an Intrusion Detection System (IDS) using control-based techniques to monitor RV’s runtime behavior and detect attacks. In addition, we show that the control-based techniques are incapable of detecting the stealthy attacks, and that the attacks can have significant adverse impact on the RV’s mission (e.g., deviate from its target significantly or result in the crash). Our findings show that using inaccurate models for invariant analysis in the case of non-linear cyber-physical systems such as RVs, opens new vulnerabilities that can be exploited to perform stealthy attacks.
View record
With the massive adoption of machine learning (ML) applications in HPC domains, the reliability of ML is also growing in importance. Specifically, ML systems are found to be vulnerable to hardware transient faults, which are growing in frequency and can result in critical failures (e.g., cause an autonomous vehicle to miss an obstacle in its path). Therefore, there is a compelling need to understand the error resilience of the ML systems and protect them from transient faults. In this thesis, we first aim to understand the error resilience of ML systems under the presence of transient faults. Traditional solutions use random fault injection (FI), which, however, is not desirable for pinpointing the vulnerable regions in the systems. Therefore, we propose BinFI, an efficient fault injector (FI) for finding the critical bits (where the occurrence of faults would corrupt the output) in the ML systems. We find the widely-used ML computations are often monotonic with respect to different faults. Thus we can approximate the error propagation behavior of an ML application as a monotonic function. BinFI uses a binary-search like FI strategy to pinpoint the critical bits. Our result shows that BinFI significantly outperforms random FI in identifying the critical bits of the ML application with much lower costs. With BinFI being able to characterize the critical faults in ML systems, we study how to improve the error resilience of ML systems. It is known that while the inherent resilience of ML can tolerate some transient faults (which would not affect the system's output), there are critical faults that cause output corruption in ML systems. In this work, we exploit the inherent resilience of ML to protect the ML systems from critical faults. In particular, we propose Ranger, a technique to selectively restrict the ranges of values in particular network layers, which can dampen the large deviations typically caused by critical faults to smaller ones. Such reduced deviations can usually be tolerated by the inherent resilience of ML systems. Our evaluation demonstrates that Ranger achieves significant resilience boosting without degrading the accuracy of the model, and incurs negligible overheads.
View record
Transient hardware faults caused by cosmic ray or alpha particle strikes in hardware components are increasing in frequency due to shrinking feature size and manufacturing variations. Fault injection (FI), where a fault is artificially introduced during a program's execution to observe its behaviour, is a commonly used experimental technique to evaluate the resilience of software techniques for tolerating hardware faults. Software-implemented FI can be performed at different levels of abstraction in the system stack, including at the compiler's intermediate representation (IR) of a program or at the assembly code level. IR-level FI has the advantage that it is closer to the source code of the program being evaluated and hence it is easier to derive insights for the design of fault-tolerance mechanisms. Unfortunately, it is not clear how accurate IR-level FI is vis-a-vis assembly-level FI, and prior work has presented contradictory findings. In this thesis, we first perform a thorough comparison study of two contradictory previous studies, and find that the inconsistent findings are due to an implementation detail regarding how candidate injection bits are selected. Further, we perform a comprehensive evaluation of the accuracy of IR-level FI across a range of benchmark programs and compiler optimization levels to supplement our findings. Our results show that IR-level FI can be as accurate as assembly-level FI for silent data corruptions (SDCs) across different benchmarks and optimization levels, but for crashes the accuracy depends on the optimization level (i.e., less accuracy when more optimizations are applied). Finally, we discuss why compiler optimizations may have an effect on the accuracy of IR-level FI for measuring crash probabilities, and present a machine learning-based technique to estimate crash probabilities that are as accurate as those measured using assembly-level FI, using only results from IR-level FI experiments. The proposed technique is shown to be capable of improving the accuracy of crash probabilities from IR-level FI by over 9 times; this allows IR-level FI to be used for accurately measuring both SDC and crash probabilities.
View record
Cyber-Physical Systems (CPSes) are being widely deployed in security- critical scenarios such as smart homes and medical devices. Unfortunately, the connectedness of these systems and their relative lack of security measures makes them ripe targets for attacks. Specification-based Intrusion Detection Systems (IDS) have been shown to be effective for securing CPSs. Unfortunately, deriving invariants for capturing the specifications of CPS systems is a tedious and error-prone process. Therefore, it is important to dynamically monitor the CPS system to learn its common behaviors and formulate invariants for detecting security attacks. Existing techniques for invariant mining only incorporate data and events, but not time. However, time is central to most CPSes, and hence incorporating time in addition to data and events, is essential for achieving low false positives and false negatives.This thesis proposes ARTINALI : A Real-Time-specific Invariant iNfer- ence ALgorIthm, which mines dynamic system properties by incorporating time as a first-class property of the system. We build ARTINALI-based Intrusion Detection Systems (IDSes) for two CPSes, namely smart meters and smart medical devices, and measure their efficacy. We find that the ARTINALI-based IDS significantly reduces the ratio of false positives and false negatives by 16 to 48% (average 30.75%) and 89 to 95% (average 93.4%) respectively over other dynamic invariant detection tools. Furthermore, it incurs about 32% performance overhead, which is comparable to other invariant detection techniques.
View record
Silent Data Corruption (SDC) is a serious reliability issue in many domains, including embedded systems. However, current protection techniques are brittle, and do not allow programmers to trade off performance for SDC coverage. Further, many of them require tens of thousands of fault injection experiments, which are highly time-intensive. In this paper, we propose two empirical models, namely SDCTune and SDCAuto, to predict the SDC proneness of a program’s data. Both models are based on static and dynamic features of the program alone, and do not require fault injections to be performed. We then develop an algorithm using both models to selectively protect the most SDC-prone data in the program subject to a given performance overhead bound. Our results show that both models are accurate at predicting the SDC rate of an application. And in terms of efficiency of detection (i.e., ratio of SDC coverage provided to performance overhead), our technique outperforms full duplication by a factor of 0.78x to 1.65x with SDCTune model, and 0.62x to 0.96x with SDCAuto model.
View record
With the projected increase in hardware error rates in the future, software needs to be resilient to hardware faults. An important factor affecting a program's error resilience is the set of optimizations used when compiling it. Compiler optimizations typically optimize for performance or space, and rarely for error resilience. However, prior work has found that applying optimizations injudiciously can lower the program's error resilience as they often eliminate redundancy in the program. In this work, we propose automated techniques to find the set of compiler optimizations that can boost performance without degrading its overall resilience. Due to the large size of the search space, we use search heuristic algorithms to efficiently explore the space and find an optimal sequence of optimizations for a given program. We find that the resulting optimization sequences have significantly higher error resilience than the standard optimization levels (i.e., O1, O2, O3), while attaining comparable performance improvements with the optimizations levels. We also find that the resulting sequences reduce the overall vulnerability of the applications compared to the standard optimization levels.
View record
Modern web applications consist of a significant amount of client-side code, written in JavaScript, HTML, and CSS. In this thesis, we present a study of common challenges and misconceptions among web developers, by mining related questions asked on Stack Overflow. We use unsupervised learning to categorize the mined questions and define a ranking algorithm to rank all the Stack Overflow questions based on their importance. We analyze the top 50 questions qualitatively. The results indicate that (1) the overall share of web development related discussions is increasing among developers, (2) browser related discussions are prevalent; however, this share is decreasing with time, (3) form validation and other DOM related discussions have been discussed consistently over time, (4) web related discussions are becoming more prevalent in mobile development, and (5) developers face implementation issues with new HTML5 features such as Canvas. We examine the implications of the results on the development, research, and standardization communities. Our results show that there is a consistent knowledge gap between the options available and options known to developers. Given the presence of knowledge gap among developers, we need better tools customized to assist developers in building web applications.
View record
While graphics processing units (GPUs) have gained wide adoption as accelerators for general-purpose applications (GPGPU), the end-to-end reliability implications of their use have not been quantified. Fault injection is a widely used method for evaluating the reliability of applications. However, building a fault injector for GPGPU applications is challenging due to their massive parallelism, which makes it difficult to achieve representativeness while being time-efficient.This thesis makes three key contributions. First, it presents the design of a fault-injection methodology to evaluate the end-to-end reliability properties of application kernels running on GPUs. Second, it introduces a fault-injection tool that uses real GPU hardware and offers a good balance between the representativeness and the efficiency of the fault injection experiments. Third, it characterizes the error resilience characteristics of twelve GPGPU applications. Last but not least, this thesis provides preliminary insights on correlations between algorithm properties and the measured silent data corruption ratesof applications.
View record
Most cloud computing clusters are built from unreliable, commercial off-the-shelf components compared with supercomputer clusters. The high failure rates in their hardware and software components result in frequent node and application failures. Therefore, it is important to understand their failures to design a reliable cloud system. This thesis presents a characterization study of cloud application failures, and proposes a method to predict application failures in order to save resources.We first analyze a workload trace from a production cloud cluster and characterize the observed failures. The goal of our work is to improve the understanding of failures in compute clouds. We present the statistical properties of job and task failures, and attempt to correlate them with key scheduling constraints, node operations, and attributes of users in the cloud. We observe that there are many opportunities to enhance the reliability of the applications running in the cloud, and further nd that resource usage patterns of the jobs can be leveraged by failure prediction techniques.Next, we propose a prediction method based on recurrent neural networks to identify the failures. It takes the resource usage measurements or performance data, and generate features to categorize the applications into different classes. We then evaluate the method on the cloud workload trace. Our results show that the model is able to predict application failures. Moreover, we explore early classification to identify failures, and find that the prediction algorithm provides the cloud system enough time to take proactive actions much earlier than the termination of applications to avoid resource wastage.
View record
Intermittent hardware faults are hard to diagnose as they occur non-deterministically. Hardware-only diagnosis techniques incur significant power and area overheads. On the other hand, software-onlydiagnosis techniques have low power and area overheads, but have limited visibility into many micro-architecturalstructures and hence cannot diagnose faults in them. To overcome these limitations, we propose a hardware-softwareintegrated framework for diagnosing intermittent faults. The hardware part of our framework, called SCRIBEcontinuously records the resource usage information of every instruction in the processor, and exposes it tothe software layer. SCRIBE has 0.95% on-chip area overhead, incurs a performance overhead of 12% and power overhead of 9%, on average.The software part of our framework is called SIED and uses backtracking from the program's crash dump to find the faulty micro-architectural resource. Our technique has an average accuracy of 84% in diagnosing the faulty resource, which in turn enables fine-grained deconfiguration with less than 2% performance loss after deconfiguration.
View record
Hardware errors are on the rise with reducing chip sizes, and power constraints have necessitated the involvement of software in hardware error detection. At the same time, emerging workloads in the form of soft computing applications, (e.g., multimedia applications) can tolerate most hardware errors as long as the erroneous outputs do not deviate significantly from error-free outcomes. We term outcomes that deviate significantly from the error-free outcomes as Egregious Data Corruptions (EDCs). In this thesis, we propose a technique to place detectors for selectively detecting EDC causing errors in an application. Our technique identifies program locations for placing high coverage detectors for EDCs using static analysis and runtime profiling. We evaluate our technique on six benchmarks to measure the EDC coverage under given performance overhead bounds. Our technique achieves an average EDC coverage of 82%, under performance overheads of 10%, while detecting only 10% of the Non-EDC and benign faults. We also explore the performance-resilience tradeoff space, by studying the effect of compiler optimizations on the error resilience of soft computing applications, both with and without our technique.
View record
Client-side JavaScript is being widely used in popular web applications to improve functionality, increase responsiveness, and decrease load times. However, it is challenging to build reliable applications using JavaScript. This work presents an empirical characterization of the error messages printed by JavaScript code in web applications, and attempts to understand their root causes.We find that JavaScript errors occur in production web applications, and that the errors fall into a small number of categories. In addition, we find that certain types of web applications are more prone to JavaScript errors than others. We further find that both non-deterministic and deterministic errors occur in the applications, and that the speed of testing plays an important role in exposing errors. Finally, we study the correlations among the static and dynamic properties of the application and the frequency of errors in it in order to understand the root causes of the errors.
View record
The scaling of Silicon devices has exacerbated the unreliability of modern computer systems, and power constraints have necessitated the involvement of software in hardware error detection. Simultaneously, the multi-core revolution has impelled software to become parallel. Therefore, there is a compelling need to protect parallel programs from hardware errors.Parallel programs’ tasks have significant similarity in control data due to the use of high-level programming models. In this thesis, we propose BlockWatch to leverage the similarity in parallel program’s control data for detecting hardware errors. BlockWatch statically extracts the similarity among different threads of a parallel program and checks the similarity at runtime. We evaluate BlockWatch on eight SPLASH-2 benchmarks to measure its performance overhead and error detection coverage. We find that BlockWatch incurs an average overhead of 15% across all programs, and provides an average SDC coverage of 97% for faults in the control data.
View record
If this is your researcher profile you can log in to the Faculty & Staff portal to update your details and provide recruitment preferences.