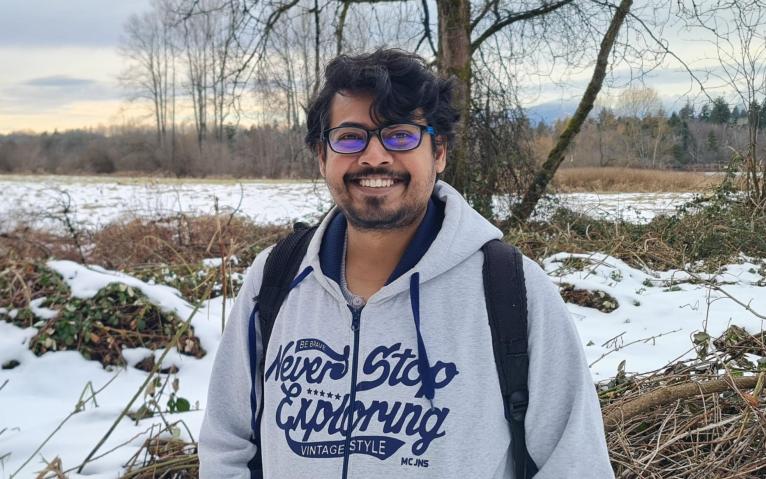
Mohit Pandey
Doctor of Philosophy in Bioinformatics (PhD)
Research Topic
Exploration of large chemical spaces with deep reinforcement and active learning
Dissertations completed in 2010 or later are listed below. Please note that there is a 6-12 month delay to add the latest dissertations.
The development of diverse cancers is often driven by the dysregulated activity of transcription factors (TFs) that control the expression of genes associated with proliferation, differentiation, and invasion. Prostate cancer (PCa) and breast cancer (BCa) are usually dependent on steroid hormone nuclear receptors signaling, specifically the androgen receptor (AR) in PCa and the estrogen receptor (ER) in BCa. This dependence has prompted the development of various hormonal therapies targeting the AR and the ER in PCa and BCa, respectively. While these treatments are initially effective, the cancer eventually recurs, with the dysregulation of additional TFs, such as Gli and N-Myc, implicated in therapeutic resistance and disease progression. This thesis aims to develop small-molecule inhibitors that interfere with crucial TF protein-protein and protein-DNA interactions, by using a combination of computer-aided drug design (CADD), cell-based characterization, and biophysical assays. We characterized a non-canonical function of the AR, where its binding to Gli facilitates the activation of Gli transcription and the progression of PCa to castration-resistant disease. Considering Gli’s role in driving cancer cell growth and the significance of AR activation in this pathway, targeting their interaction would provide a new strategy to inhibit PCa progression. Our work on N-Myc, a TF long deemed “undruggable,” led to the development of a first-in-class inhibitor (VPC-70619) that prevents its binding to DNA E-boxes, leading to decreased proliferation of N-Myc driven cancer models. Finally, advanced BCa therapies like antiestrogens, target the estrogen binding site in ER, but commonly fail due to resistance. By targeting the interaction between ER and its coactivators at an allosteric site, we identified a compound (VPC-260724) that overcomes therapeutic resistance and inhibit the growth of tamoxifen resistant BCa models. Our exploration of TF interactions, namely those involving ER, AR, Gli, and N-Myc, not only sheds light on the molecular mechanisms driving PCa and BCa, but also lays the foundation for the development of innovative therapeutic strategies. The design of small molecules through CADD and robust experimental validation offers a promising approach to revolutionizing the landscape of cancer therapeutics and to develop more effective targeted treatments against advanced stages of these diseases.
View record
Breast cancer (BCa) is a significant cause of cancer-related deaths among females globally. Approximately 45% of BCa patients develop treatment resistance or suffer from a lack of targeted therapies. Previously, we have shown that Semaphorin 3C (SEMA3C) is an autocrine growth factor that drives the growth and treatment resistance of various cancers. This study aims to investigate the functional role of SEMA3C in breast cancer progression and treatment resistance. My analysis of clinical datasets revealed elevated levels of SEMA3C mRNA in breast tumors compared to normal adjacent tissue. SEMA3C expression was positively correlated with the expression of various oncogenes implicated in breast cancer. Both estrogen receptor (ER) positive (ER⁺/HER2ˉ) BCa and triple negative breast cancer (TNBC) cells exhibited higher levels of SEMA3C protein compared to a non-cancer mammary epithelial cell line (MCF10A). Stimulation with recombinant SEMA3C activated EGFR, MAPK and AKT signaling in both ER⁺ and TNBC cells. Conversely, SEMA3C silencing inhibited ER expression, EGFR, MAPK, and AKT signaling, while inducing apoptosis. Silencing of SEMA3C significantly suppressed the growth of ER⁺ BCa and TNBC cells, indicating a growth dependency on SEMA3C. Tamoxifen-resistant cells (TamC3, TamR3) remained reliant on SEMA3C for growth and survival, suggesting its persistence in treatment resistance. Additionally, SEMA3C suppression enhanced the efficacy of certain chemotherapies and targeted therapies in TNBC cells. Additionally, treatment with SEMA3C pathway inhibitors, B1SP (Fc fusion protein) and ALS, attenuated SEMA3C-induced signaling and growth in both ER⁺ and TNBC cells. This study highlights the functional role of SEMA3C in breast cancer signaling and growth, suggesting its potential as a therapeutic target. Targeting SEMA3C may offer benefits in improving treatment outcomes for breast cancer patients, particularly in cases of endocrine treatment resistance and TNBC.
View record
“Undruggable” or “difficult to drug” targets make up most of the human proteome; these proteins are described as such when it is considered impossible to pharmacologically target them. Therefore, “undruggable and difficult to drug” proteins represent significant challenges to the established drug discovery pipelines, but their successful inhibition would enable access to a wider range of therapeutic opportunities.In this thesis, we utilized various in silico tools to identify and design small molecule drug prototypes that could inhibit such undruggable and difficult targets. First, we introduced the recent development of new computer-aided drug design (CADD) methodologies and tools such as consensus docking, and Deep Docking. We then described the deployment of these innovative CADD methodologies to discover novel small molecule therapeutics against considered-as-difficult targets such as N-Myc, SARS-CoV-2 PLpro and Mpro. N-Myc is a highly desirable oncoprotein involved in many cancers and there is significant interest for targeting N-Myc in prostate cancer (PCa) and particularly in neuroendocrine prostate cancer (NEPC, an advanced, low-survival stage of PCa). However, N-Myc is considered an undruggable and unsuitable for small molecule inhibition due to its overall disordered structure. Thus, we developed new N-Myc specific small molecules with established and newly developed CADD protocols. Other examples of high value, but challenging targets are the viral main protease (Mpro) and the papain-like protease (PLpro) from severe acute respiratory syndrome-related coronavirus-2 (SARS-CoV-2), as they are central pieces in its replication-transcription complex. However, intrinsic flexibility, multiple protonation state, solvent-exposed nature and other active site features unique to each protease decrease the success rate of conventional drug discovery protocols. Therefore, we discussed the identification of Mpro hits through naïve large virtual screening and ultra-large virtual screening that incorporated advanced consensus approaches. We also recapitulated the identification of new PLpro inhibitors through fine-tuned pharmacophore modelling and large-scale virtual screening with Deep Docking. In this thesis, we identified and designed small molecule inhibitors for each mentioned target of interest using state-of-the-art CADD methodologies. Notably, the compounds presented in this thesis provide the initial blueprints for the potential development of new anticancer and antiviral drugs.
View record
Prostate cancer is one of the leading causes of cancer-related death in men worldwide. If diagnosed early, prostate cancer can be treated by surgery and/or radiotherapy. In cases where the cancer has returned or is more aggressive and has metastasized, hormone therapy is the standard treatment. While initially effective, resistance to hormone therapy often occurs. Therefore, there is a pressing demand for new therapeutics to be developed to treat this disease. Previous studies have established that in up to 50% of all prostate cancer cases, a genomic irregularity involving the ETS-related gene (ERG) is present. This alteration results in the aberrant production of predominantly amino-terminal truncated ERG proteins in the prostate where it is linked to disease development and progression. This thesis tested the hypothesis that direct, small molecule targeting of ERG DNA binding could result in inhibition of the metastatic potential of PCa through the following specific aims: a) develop and apply in vitro assays to validate inhibitory activities/mechanisms of lead anti-ERG compounds, and b) determine the therapeutic effects of the lead compounds based on their effects and activity in in vivo xenograft models. The results demonstrate the direct binding of a novel small molecule, VPC-18005, with the ERG-ETS domain using biophysical approaches. This was further supported by reduced migration and invasion rates of ERG expressing prostate cancer cells, and reduced metastasis in a zebrafish xenograft model following exposure to VPC-18005. These results support the concept that small molecules targeting the ERG-ETS domain that suppress transcriptional activity and reverse transformed characteristics of prostate cancers aberrantly expressing ERG can be developed. It is hoped that these approaches might lead to identification of small molecules that can be further developed as drug candidates as alternatives to, or in combination with, current therapies for prostate cancer patients harboring ERG fusions.
View record
To date, castration-resistant prostate cancer (CRPC) remains an incurable disease, as conventional therapeutic inhibition of androgen receptor (AR) signaling with anti-androgens inevitably leads to treatment-resistance, further progression to final stage neuroendocrine prostate cancer (NEPC), and rapid demise. Therefore, development of novel targeted therapies for CRPC and NEPC is of paramount importance.Targeting the oncogenic activity of Myc family of transcription factors has long been and currently is a major topic of cancer research. While Myc is an essential regulator of normal growth, its exacerbated expression is a hallmark of human cancer. Amplifications of Myc family members play critical roles in prostate cancer progression and therapy-resistance. c-Myc is amplified across its full-spectrum and has special relevance in CRPC as it positively regulates the expression and activity of AR itself as well as of ligand-independent AR-V truncated variants, such as AR-V7 that confers resistance to anti-androgens. Moreover, N-Myc amplifications induce NEPC phenotype.Although Myc proteins are high-value targets for therapeutic intervention, clinically viable Myc-directed inhibitors await discovery. Intrinsically disordered, Myc lacks effective binding pockets. Therefore, the use of conventional methods of structure-based drug discovery is an inherent challenge. Moreover, the oncogenic function of Myc is dependent on its dimerization with the obligate partner Max, which together form a functional transcriptional complex capable of activating critical genomic targets and eliciting oncogenic effects.This dissertation describes the discovery and development of novel small-molecule inhibitors targeting the oncogenic activity of Myc-Max complexes. Specifically, we utilized methods of computer-aided drug discovery (CADD) to target directly complexes of c-Myc and N-Myc with Max, as well as Myc-upregulated hnRNP A1 splicing factor. The use of CADD enabled us to identify small-molecule drug candidates, which selectively disrupt critical protein-nucleic acid interactions – a therapeutic approach that has not been previously exercised for these targets. The CADD techniques encompassed large-scale structure-based docking and molecular dynamics simulations along with ligand-based approaches including pharmacophore modeling, chemical similarity searches and ADMET profiling, complemented by experimental validation. On the outlook, the identified lead inhibitors lay the foundation for development of safe and effective clinical candidates that may serve as prospective therapeutics for CRPC and NEPC.
View record
Estrogen receptor alpha positive (ERα+) disease constitutes approximately 75% of all breast cancer (BCa) cases. However resistance to hormone therapy is observed in early-stage as well as in metastatic disease. Importantly, 70% of ERα+ primary tumors retain active ERα when they metastasize and, therefore, ERα continues to play a role in the resistant form of the disease. Moreover, the effectiveness of conventional hormone therapies is hampered due to gain-of-function mutations that may render the receptor constitutively active. Thus drugs that target the ERα estrogen binding site can become ineffective with time. Moreover, cross-talk between ERα and activated growth factor receptors, or their downstream kinases have shown to play a major role in activating ERα even in the absence of estradiol. Taken together, these observations highlight the importance of developing therapeutics that target alternative sites on the receptor, for instance, those that directly act on the co-activator binding pocket called activation function-2 (AF2) site. Using methods of in-silico screening followed by a systematic computer-guided lead optimization process, we were able to develop several promising small-molecule inhibitors that target the AF2 functional site of ERs. This thesis describes the establishment of an experimental pipeline and development of such inhibitors. The identified lead compound VPC-16606 effectively blocked ERα-co-activator interactions, demonstrated a strong anti-proliferative effect against a panel of ERα+ cells including Tamoxifen-resistant cells and down-regulated ERα-dependent genes. Most importantly, VPC-16606 successfully inhibited known constitutively active mutant forms of ERα observed in clinical settings where BCa patients have relapsed on aromatase inhibitors. Furthermore, the compound also reduced tumor burden in vivo. Overall, these studies helped to identify a novel class of ERα AF2 inhibitors which have the potential to effectively inhibit ERα activity by a unique mechanism and to circumvent the issue of hormone resistance in BCa patients.
View record
Interest in developing androgen receptor (AR) inhibitors with novel mechanism of action for the treatment of prostate cancer (PCa) is on the rise since the commercial anti-androgens (including recently approved drug, Enzalutamide) face clinical limitations. Current therapies fail over a period of time because they all target mutation-prone androgen binding pocket on AR to which the receptor has already developed effective resistance mechanisms. Hence, there is a pressing need for novel therapeutics that inhibit the AR through alternative modes of action. To address this problem, we have used in silico drug design methodology to create new drugs that act on an entirely different site on the AR, a recently identified co-activator site called binding function-3 (BF3). This dissertation describes the discovery and development of novel anti-androgens directed towards the BF3 surface of the AR. These inhibitors were developed through a series of computational experiments followed by extensive biological validations. Based on the activity profile of the identified inhibitors, it can be anticipated that these drug prototypes will lay a foundation for the development of alternative or supplementary small-molecule therapies capable of combating PCa even in its drug resistant forms. Because the emergence of castration resistance is the lethal end stage of the disease, we anticipate that the thesis work will eventually have a substantial impact on the survival of prostate cancer patients.
View record
Prostate cancer (PCa) is the most commonly diagnosed cancer in men, and the second leading cause of male cancer death in North America. The androgen signalling pathway plays a central role in the development and advancement of PCa as well as in its progression to a lethal castration-resistant stage (CRPC). The human androgen receptor (AR) is a master regulator of PCa progression and survival, and a well-validated drug target for PCa. All clinically used AR inhibitors (antiandrogens) are initially effective to PCa; however, they invariably cause resistance. Thus, there is a continuing need for developing novel anti-AR drugs for the treatment of PCa and CRPC. Although the mechanism of resistance to antiandrogens is not completely clear, it involves mutation-driven antagonist-to-agonist transformation of the AR response, and the emergence of AR splice variants (ARVs) lacking the entire ligand-binding domain (LBD) of the protein. This dissertation describes the discovery and development of novel AR inhibitors directed towards the conventional androgen binding site (ABS) of the receptor, as well as the discovery of an entirely novel class of inhibitors targeting the DNA-binding domain (DBD) of the AR. Both types of AR inhibitors were identified through virtual screening and molecular modeling, followed by in vitro and/or in vivo validation of developed drug prototypes. The objective of developing novel chemotypes for ABS binders and AR DBD inhibitors is to help circumvent drug resistance problem in the field of PCa.
View record
Infectious diseases caused by bacterial pathogens continue to be major public health concerns affecting millions of human lives annually, as conventional treatment via antibiotics has lost its effectiveness due to growing problems of drug resistance. Recent advancements in systems biology, high-throughout sequencing, protein interaction study and computer-aided drug development can offer possible solutions to antibiotic resistance through discovery of novel antimicrobials. The thesis describes several bioinformatics approaches that focus on protein interaction network (PIN) studies, analyses of targetable protein indels (insertions and deletions) and virtual compound screening for new antibacterial candidates – approaches integrated into an antibiotic discovery pipeline for methicillin-resistant Staphylococcus aureus (MRSA252). In the course of the described work we identified new drug targets corresponding to highly interacting proteins (hubs) through comprehensive PIN analysis in MRSA252. The advantage of using hub proteins as targets is established by their essentiality, non-replaceable PIN position and lower rate of mutation, all of which can help to counter bacterial resistance. To accelerate these studies hub predicting tools have been developed to assist proteomics experiments for PIN discovery and to facilitate drug target identification in pathogens. Because some bacterial proteins are conserved in humans, we applied the indel (insertion or deletion) concept to locate unique compound-binding sites that enabled us to specifically target conserved and essential bacterial hubs. We demonstrated associations between the presence of sizable indels in proteins with their essentiality and network rewiring capability, which established indels as potential markers for drug targets. To provide the research community a fast and user-friendly web portal for identification and characterization of indel-bearing drug targets, the Indel PDB database has been developed to characterize the functional and structural features of 117,266 indel sites across numerous species. Finally, combining the above bioinformatics methodologies with a rapid and efficient procedure of virtual screening allowed discovery of compounds that effectively inhibited MRSA252 cell growth with no signs of human toxicity. We anticipate that the drug discovery pipeline along with established MRSA PIN resource, hub prediction tools and indel database will provide a framework for the development of next-generation antibiotics in other existing or emerging pathogens.
View record
The emergence of pathogens resistant to available drug therapies is a pressing global health problem. Antimicrobial peptides (AMPs) may potentially form new therapeutics to counter these pathogens. AMPs are key components in the mammalian innate immune system and are responsible for both direct killing and immunomodulatory effects in host defense against pathogenic organisms. This thesis describes computational methods for the identification of novel natural and synthetic AMPs. A bioinformatic resource was constructed for classification and discovery of gene- coded AMPs, consisting of a database of clustered known AMPs and a set of hidden Markov models (HMMs). One set of 146 clusters was based on the mature peptide sequence, and one set of 40 clusters was based on propeptide sequence. The bovine genome was analyzed using the AMPer resources, and 27 of the 34 known bovine AMPs were identified with high confidence and up to 69 AMPs were predicted to be novel peptides. One novel cathelicidin AMP was experimentally verified as up-regulated in response to infection in bovine intestinal tissue. A chemoinformatic analysis was performed to model the antibacterial activity of short synthetic peptides. Using high-throughput screening data for the activities of over 1400 peptides of diverse sequence, quantitative structure-activity relation (QSAR) models were created using artificial neural networks and physical characteristics of the peptide that included three-dimensional atomic structure. The models were used to predict the activity of a set of approximately 100,000 peptide sequence variants. After ranking the predicted activity, the models were shown to be very accurate. When 200 peptides were synthesized and screened using four levels of expected activity, 94% of the top 50 peptides expected to have the highest level of activity were found to be highly active. Several promising candidates were synthesized with high quality and tested against several multi- antibiotic-resistant pathogens including clinical strains of Pseudomonas aeruginosa, Staphylococcus aureus, Enterococcus faecalis and Escherichia coli. These peptides were found to be highly active against these pathogens as determined by minimal inhibitory concentration; this serves as independent confirmation of the effectiveness of high-throughput screening and in silico analysis for identifying peptide antibiotic drug leads.
View record
Theses completed in 2010 or later are listed below. Please note that there is a 6-12 month delay to add the latest theses.
The rapid global spread of the SARS-CoV-2 virus facilitated the development of novel direct-acting antiviral agents (DAAs) targeting the virus’s essential proteins, such as Papain-like protease or PLpro. This enzyme plays a dual role participating in the maturation of viral proteins and in the suppression of the host immune system. In this work, we performed a virtual screening of ultra-large chemical libraries to identify prospective non-covalent PLpro inhibitors. The analysis of active compounds revealed their somewhat limited diversity, which is a recurring theme across publications on the discovery of PLpro ligands. This is likely attributed to the induced-fit nature of the enzyme’s active site which limits the effectiveness of rigid molecular docking protocols. Even with such constraint, we demonstrate that the identified compound VPC-300195 ranks among the top non-covalent PLpro inhibitors discovered through in silico methodologies and reported to date. After discovering an initial set of promising compounds through virtual screening, we developed a deep reinforcement learning-based approach to further optimize the hit molecules. This method modifies the candidate molecule inside of the protein pocket using fragment-based addition and replacement. We optimized the series of VPC-300195 derivatives on multiple parameters including Quantitative Estimate of Druglikeness (QED), Synthetic Accessibility (SA) score, predicted toxicity, and binding free energy. The state of the newly elaborated molecule was estimated using Molecular Mechanics Generalized Born Solvent Accessible Surface Area (MMGBSA) while performing complementary minimization (taking into account the solvent effects and protein dynamics).Taken together, the results of this work laid a foundation for the development of effective non-covalent SARS-CoV-2 inhibitors and provided a potential platform for effective optimization of initial hit compounds using methods of reinforcement learning.
View record
Severe Acute Respiratory Syndrome Coronavirus 2 (SARS-CoV-2) caused a global healthcare crisis due to COVID-19 pandemic. In absence of efficacious antiviral drugs against this new Coronavirus species, scientific and clinical efforts initially focused on identifying repositionable FDA-approved drugs to be rapidly deployed worldwide. As the efforts shifted to identifying novel antiviral drugs specific to SARS-CoV-2, while vaccine development took place, computer-assisted drug discovery (CADD) screening strategies, predominantly through molecular docking, emerged as versatile, fast, and economic means to prioritize novel and promising compounds that could progress swiftly to clinical validation. Alternative to docking, are scoring functions (SFs) inferred from machine learning (ML) models. Such models are trained on protein-ligand interactions and can be used to predict binding affinity of novel molecules against a target of interest. The first chapter of this thesis discusses computational methods used throughout this work focused on CADD enabled identification of novel direct acting antivirals (DAA) for SARS-CoV-2pathogen. The second chapter reconciliates numerous SARS-CoV-2 repurposing studies while underscoring discrepancies, mapping drug ontologies and highlighting repositionable drugs with the highest agreement among the studies. Importantly, we experimentally validate those candidate drugs – reporting still divergent potency values. We conclude with describing a sustainable route of drug repurposing based on the collective findings. The third chapter, on the other hand, focuses on the shift towards de-novo drug discovery through molecular docking of screening compoundsagainst the main protease (Mpro) enzyme of the SARS-CoV-2. Therein, we utilized five different computational protocols and employed a manifold of hit-diversifying filtering strategies on a record-breaking database of 40 billion molecules. The ultra-large screening resulted in the discovery of two promising noncovalent compounds for translational development. Finally, in the fourth chapter of the thesis, we describe a proof of concept of a new SF alternative to molecular docking consisting of a graph neural network (GNN) model with residual skip connections and attention-based graph pooling. We capitalize on the reported results from the third chapter’s for benchmarking the model’s performance. We report a state-of-the-art performance of the proposedmodel (Pearson > 0.8 across DAVIS, KIBA, BindingDB and PDBBind databases).
View record
The full abstract for this thesis is available in the body of the thesis, and will be available when the embargo expires.
View record
Modern drug discovery is broadly facilitated by the use of various computational tools and techniques. These enable to significantly reduce the cost and amount of time required to identify drug candidates. The development of cancer therapeutics has particularly benefited from the rise of the field of computer-aided drug design (CADD). Herein we present several practical examples of the development of small molecule-inhibitors of three types of cancer drug targets (protein-protein, protein-DNA and protein-RNA interaction interfaces) with the use of CADD tools.First, a protein-DNA interaction targeting is demonstrated with the case of DNA Topoisomerase II (TopoII). In Chapter II, a comprehensive overview of computational studies on the development of TopoII inhibitors is provided. We then discuss computational validation of the proposed mode of action and optimization of a promising catalytic inhibitor of TopoII called T60.Second, we identified inhibitors of an RNA-protein interaction Lin28-let-7. The virtual screening used in this work resulted in a particularly high 18% hit-rate supporting the utility of CADD methodology.Third, we employed homology modelling and virtual screening to discover inhibitors of a protein-protein complex, Siah1-UBC13. The hit molecule exhibited a similar activity profile to a drug candidate that was previously found in an in vitro screening of several thousand chemicals. This exemplifies the cost efficiency of in silico screens compared to in vitro.Altogether, the presented results demonstrate the broad utility of CADD methodology in precision cancer drug discovery.
View record
Computer Aided Drug Discovery (CADD) is a broad field which uses scientific tools from various disparate disciplines towards drug discovery and design. The tools of CADD include receptor based methods such as docking and Molecular Dynam- ics (MD), and ligand based methods such as Quantitative Structure Activity Rela- tionship (QSAR) / Quantitative Structure Property Relationship (QSPR) approaches relying directly on the structure of small molecules. In this thesis both of these approaches to CADD have been utilized together to drug topoisomerases. There are two clinically relevant members of the Topoisomerase (TOP) protein family, TOP I and TOP II, both of which the tools of CADD were applied. For the former, sev- eral novel TOP I natural product-like inhibitors were developed and characterized with molecular dynamics simulations. This led to the in-silico based prediction of unique but strong non-covalent interactions in the binding site, as well as a rational- ization of the difference in activity between two enantiomers. For TOP II, a broader CADD campaign was initiated to screen ⇠ 12 million molecules from the ZINC Is Not Commercial (ZINC)-15 [1] database against TOP II. This was facilitated by the implementation of consensus voting protocols from various virtual screening programs [2] and compositions of machine learning techniques. With a synergy between in-silico and wet-lab components, a rational drug discovery pipeline was executed to discover and characterize a highly potent inhibitor. The identified com- pound has been shown to inhibit topoisomerase in a Kinetoplast DNA (KDNA) de- catenation assay with a nanomolar Concentration at 50% Cellular Inhibition (IC50). Furthermore, it has been demonstrated that the identified drug candidate does not act as a ”DNA poison”, as no linear Deoxyribonucleic Acid (DNA) is formed upon incubation with TOP II in a relaxation assay and no general DNA damage is observed. Finally, a mechanism of action for the lead compound is proposed, based on experimental and in-silico evidence.
View record
Motivation: Recent advances in the areas of bioinformatics and predictive chemogenomics are poised to accelerate the discovery of small-molecule modulators of cellular processes. In that regard, combining large genomics and molecular data sources with recently emerged powerful deep learning techniques has the potential to revolutionize predictive biology. In this study, we present Deep Compound Profiler (DeepCOP), a deep learning (DL) based approach that can predict gene regulating effects of chemicals. This model, among many other potential applications, can be used for direct identification of a drug candidate causing a desired gene expression response, without utilizing any information on its interactions with particular protein target(s).Results: In this study we successfully combined molecular fingerprint descriptors and gene descriptors (derived from GO terms) to train deep neural networks (DNNs) that predict differential gene regulation endpoints collected in the Library of Integrated Network-Based Cellular Signatures (LINCS) database. The developed models yielded 10-fold cross validation AUC values of and above 0.80, as well as enrichment factors of >5. We validated the developed models using an external RNA-Seq dataset generated in-house that described the effect of three potent antiandrogens (with different modes of action) on gene expression in LNCaP prostate cancer cell line. The results of this pilot study demonstrate that DL models can effectively synergize molecular and genomic descriptors and can be used to screen for novel drug candidates with the desired effect on gene expression. We anticipate that such models can find a broad use in developing novel cancer therapeutics and can facilitate precision oncology efforts.
View record
Drug discovery is a rigorous process that can cost up to 3 billion dollars and takes more than 10 years to bring new therapeutics from bench to bedside. While virtual screening (such as molecular docking) can significantly speed up the discovery process and improve hit rates, its speed already lags behind the rate of the explosive growth of publically available chemical databases which already exceed billions of entries. This recent surge of available chemical entities presents great opportunities for discovering novel classes of small molecule drugs but also brings a significant demand for faster docking methods. In the current thesis, we illustrated the need for a faster screening method by virtually screening 7.6 million molecules against Thymocyte selection-associated high mobility group box protein (TOX). Then we demonstrated that the deep learning-based method of ‘Progressive Docking (PD2.0)’ can speed up such virtual screening by up to hundred folds. In particular, by utilizing deep learning QSAR models trained on the docking scores of a subset of the database, one can approximate in an iterative manner the docking outcome of unprocessed entries. We tested the developed method against various targets including ETS transcription factor ERG, Estrogen Receptor Activation Function 2 (ERAF2), Androgen Receptor (AR), Estrogen Receptor (ER), Sodium-Ion Channel (Nav1.7) and many more. In this work, we identified 18 active compounds against TOX with micro-molar potency. We also used the PD2.0 method to dock up to 1.3 billion compounds from the ZINC15 database and demonstrated that this deep-learning-based approach resulted in 65X speed acceleration and 130X Full Predicted Database Enrichment (FPDE) while retaining more than 90% of good hits. We also demonstrate the method’s robustness by docking 570 million compounds from the ZINC15 database into 13 diverse drug targets including ERG.
View record
The human Androgen Receptor (AR) is a ligand-activated transcription factor that plays a pivotal role in the development and progression of prostate cancer (PCa). AR is also critical for the survival of many forms of castration resistant prostate cancer (CRPC). The currently used AR inhibitors (anti-androgens) face clinical limitations as drug resistance has been reported in patients, both primary and acquired. In 20% of the CRPC patients resistance to AR antagonists arise due to the mutations in the androgen binding site (ABS) of the receptor. Some mutations can convert antagonist to agonist. Such gain-of-function mutations have been reported across the length of the ligand binding domain (LBD) of AR that contains the ABS, it is imperative to develop a prognostic personalized therapy platform which would equip clinicians with actionable strategies in regard to previously unreported AR aberrations when they are encountered in clinical samples. The goal of this study is to develop a theoretical approach that can characterize such previously unreported AR mutants and predict their response to the currently used anti-androgens. Thus, a novel ‘in-silico’ pipeline has been created that amalgamates the state-of-the-art cheminformatics methods with experimental assays that enable predicting AR mutants and characterizing their drug responses with high accuracy. The corresponding pipeline utilizes QSAR approach that extracts key protein-ligand interactions quantified by the in-house developed 4D-inductive molecular descriptors. The developed QSAR models reach about 90% accuracy that forecasts agonist or antagonist behaviors of AR mutants caused by clinically used and experimental anti-androgens. Furthermore, a previously unreported mutant, T878G has been predicted to be activated by both first and second generation anti-androgens and the corresponding experimental evaluation confirmed this prediction. Finally, the applicability and adaptability of the developed cheminformatics pipeline was tested against an experimental anti-androgen drug ODM-201 which was not a part of the QSAR training dataset, and the predictions were confirmed by experimental evaluations. Overall, the developed pipeline can provide useful insights towards understanding the changing genomic landscape of advanced PCa.
View record
Androgen receptor (AR) plays a critical role in prostate cancer development and progression. Allcurrent therapeutic AR inhibitors modulate the receptor via direct binding to its HormoneBinding Site (HBS). Despite the identification of other small molecule binding areas on the ARsurface including Activation Function 2 (AF2), binding function 3 (BF3), and N-terminal domain(NTD), HBS continues to be the major target site for AR antagonists (even though this site isprone to resistant mutations). Thus, there is a high need for the identification and development ofnovel antagonists targeting HBS of the AR.In this study, an effective QSAR modeling pipeline was set up and proved to be capable ofidentifying new AR antagonists from a large ZINC collection of purchasable chemicals. Inparticular, we have utilized DRAGON, INDUCTIVE and MOE descriptors to create variousbinary QSAR models of anti-AR activity. When we have applied the developed QSAR solutionsto screen more than 2 million chemicals from the ZINC database, we were able to identify 39potential candidate AR HBS binders. When they were tested in the DHT displacement assay, 9chemicals demonstrated the corresponding IC₅₀ values in efficient low-micromole range. Ofthose, 9 compounds later exhibited ability to inhibit AR in the eGFP transcriptional assay withthe IC₅₀ values established at 1.04-16.18 μM level. Notably, 6 discovered chemicalsdemonstrated concentration-dependent suppression of survival of LNCaP prostate cancer celllines.The results of this study set a ground for the development of an entire novel chemical class ofAR antagonists that are distinct for the currently marketed drugs such as Nitalutamide,Flutomide, Cassodex, and MDV3100 that all share significant structural similarity.
View record
If this is your researcher profile you can log in to the Faculty & Staff portal to update your details and provide recruitment preferences.