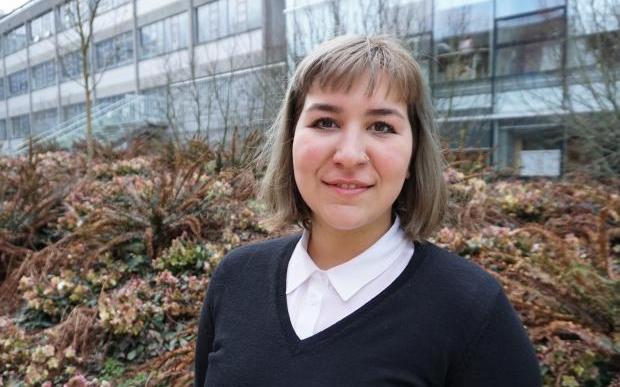
Delaram Behnami
Doctor of Philosophy in Electrical and Computer Engineering (PhD)
Research Topic
A machine learning framework for automated diagnostic assistance in echocardiographic images
Purang Abolmaesumi received his BSc (1995) and MSc (1997) from Sharif University of Technology, Iran, and his PhD (2002) from UBC, all in electrical engineering. From 2002 to 2009, he was a faculty member with the School of Computing, Queen’s University. He then joined the Department of Electrical and Computer Engineering at UBC, where he is a Canada Research Chair, Tier II, in Biomedical Engineering and a Professor, with Associate Membership to the Department of Urologic Sciences.
Dr. Abolmaesumi is internationally recognized and has received numerous awards for his pioneering developments in ultrasound image processing, image registration and image-guided interventions. He is the recepient of the Killam Faculty Research Prize at UBC. He currently serves as an Associate Editor of the IEEE Transactions on Medical Imaging, and has served as an Associate Editor of the IEEE TBME between 2008 and 2012. He is a Board Member of the International Society for Computer Aided Surgery, and serves on the Program Committees of the Medical Image Computing and Computing and Computer Assisted Intervention (MICCAI), International Society for Optics and Photonics (SPIE) Medical Imaging, and the International Conference on Information Processing in Computer Assisted Interventions (IPCAI). Dr. Abolmaesumi is the General Chair of IPCAI 2014 and 2015, and has served as Program Chair of IPCAI 2012 in Pisa and Workshop and Tutorial Chair of MICCAI 2011 in Toronto.
We are actively looking for individuals with strong mathematical, computer science, and engineering background with interest in machine learning applications in biomedical engineering and medical imaging.
G+PS regularly provides virtual sessions that focus on admission requirements and procedures and tips how to improve your application.
These videos contain some general advice from faculty across UBC on finding and reaching out to a potential thesis supervisor.
Dissertations completed in 2010 or later are listed below. Please note that there is a 6-12 month delay to add the latest dissertations.
Heart failure (HF) is associated with poor patient outcomes and burdens healthcare systems and clinicians. Fortunately, therapeutic options are available for managing cardiac dysfunction if diagnosed early. Echocardiography (echo) can be used to assess cardiac function swiftly and detect signs or risk factors of HF. Nonetheless, echo acquisition and interpretation require extensive training and experience, leading to exceeding demand for the available clinical echo services. This thesis investigates the feasibility of machine learning (ML)-based solutions for analyzing heart function based on clinical echo data and available annotations. The goal is to automate measurements of indicators of functional diseases. We focus on guideline-aware supervised learning frameworks for assessing LV ejection fraction (EF), regional wall motion abnormality (WMA), and LV diastolic dysfunction (LVDD). We propose spatio-temporal neural networks to determine EF from echo cine loops. We utilize multi-task learning with observer variability modelling is leverage the label noise and decouple errors in different available EF labels. In the context of regional systolic function, we present an error quantification and visualization framework to evaluate the generalizability of disease-agnostic models on diseased cohorts. We validate segmentation models trained on standard populations in a WMA cohort and report global and local error metrics with weak wall segment labels. This framework enables us to further identify failure modes in trained ML models. Using the errors obtained from the weak labels, we observed that segmentation performance might become jeopardized in the presence of akinetic LV wall segments. Finally, in the most extensive study of its kind, we demonstrate the impacts of the updated clinical guidelines for diastolic function assessment based on measurements derived from echo. We propose a neural network to replicate the latest clinical guidelines for diastolic function classification and extend this model to a regression framework to obtain a novel continuous LVDD scoring system. Increasing the size and diversity of the training and test set for model training and clinical validation is critical to further developing ML-driven heart disease diagnostic tools. Future work may involve ML-based multi-chamber quantification, myocardium localization, and Doppler image analysis toward automatic disease diagnosis.
View record
Ultrasound-guided needle biopsy with pathologic grading is the standard-of-careto guide the systematic biopsy of the prostate. Systematic transrectal ultrasound isblind to prostate pathology; and diagnostic accuracy is still one of the main clinicalchallenges in prostate cancer treatment and management. Hence, there is a clearneed for improving US data and providing solutions to guide the biopsy procedure.Several methods including temporal enhanced UltraSound have been proposed toimprove US-based tissue typing. The ultimate clinical goal is to display the cancerlikelihood maps on B-mode ultrasound images in real time to help with indicationof cancer in the core, decision support for biopsy targeting, and eventually reduc-ing the number of unnecessary biopsies. The objective of this dissertation is todeploy this by integration of temporal enhanced UltraSound and machine learningapproaches. Towards fulfilling this objective, in this dissertation a weakly super-vised learning technique is utilized to learn from ultrasound image regions associ-ated with the corresponding data on patient level. To improve the prostate cancerdetection we consider the nonstationarity nature of the data and use a complexneural network to find a better representation of the data in the embedding spacefor a better classification. Later, we automate reliable detection by estimatingthe model and label uncertainty. We finally show in order to improve the performanceof prostate cancer classification, the label noise needs to be considered and in thiswork, the latter is done by implementing a label refinement technique.
View record
Echocardiography (echo) plays an important role in cardiac imaging and provides a non-invasive, low-cost, and widely available diagnostic tool for the comprehensive evaluation of cardiac structure and function. However, ultrasound image interpretation remains a challenge, particularly for the novices. In this thesis, I propose several machine learning methods with the aim of helping in cardiac assessment. In particular, the proposed methods take advantage of novel advancements in spatio-temporal analysis of videos to find a better representation of the cardiac cine series.First, I propose a customized supervised learning method in order to find two specific phases in a cardiac cycle. Specifically, identification of the end-systolic (ES) and end-diastolic (ED) phases from the echo cine series is a critical step in the quantification of cardiac chamber size and function. Later, I develop a self-supervised learning method for frame-rate up-conversion to augment conventional imaging without the need of specialized beamforming and imaging hardware. In another work, I propose a self-supervised learning framework to synchronize various cross-sectional 2D echo videos without any human supervision or external inputs. I show that such rich, yet free semantic representation can be used not only for synchronization of multiple cine series, but also for fine-grained cardiac phase detection. Finally, I propose a semi-supervised learning method to detect the cardiac rhythm based solely on echo without the need for an electrocardiogram (ECG), which is commonly used for cardiac rhythm detection.
View record
The continuous advent of novel imaging technologies in the past two decades has created new avenues for biomechanical modeling, biomedical image analysis, and machine learning. While there still is relatively a long way ahead of the biomedical tools for them to be integrated into the conventional clinical practice, biomechanical modeling and machine learning have shown noticeable potential to change the future of treatment planning. In this work, we focus on some of the challenges in the modeling of the masticatory (chewing) system for the treatment planning of jaw reconstructive surgeries. Here, we discuss novel methods to capture the kinematics of the human jaw, fuse information in between imaging modalities, estimate the missing parts of the 3D structures (bones), and solve the inverse dynamics problem to estimate the muscular forces. This research is centered around the human masticatory system and its core component, the mandible (jaw), while focusing on the treatment planning for cancer patients. We investigate jaw tracking and develop an optical tracking system using subject-specific dental attachments and infrared markers. To achieve that, a fiducial localization method was developed to increase the accuracy of tracking. In data fusion, we propose a method to register the 3D dental meshes on the MRI of the maxillofacial structures. We use fatty ellipsoidal objects, which resonate in MRI, as fiducial landmarks to automate the entire workflow of data fusion. In shape completion, we investigate the feasibility of generating a 3D anatomy from a given dense representation using deep neural architectures. We then extend on our deep method to train a probabilistic shape completion model, which takes a variational approach to fill in the missing pieces of a given anatomy. Lastly, we tackle the challenge of inverse dynamics and motor control for biomechanical systems where we investigate the applicability of reinforcement learning (RL) for muscular force estimation. With the mentioned portfolio of methods, we try to make biomechanical modeling more accessible for clinicians, either via automating known manual processes or introducing new perspectives.
View record
Heart disease is one of the foremost causes of mortality worldwide. Echocardiography (echo) is a commonly used modality to study the heart's structure and function as it is non-invasive and cost-effective. In recent years, the emergence of readily accessible ultrasound (US) devices, namely point-of-care ultrasound (POCUS), has accelerated the widespread uptake of echo. However, robust echo acquisition and interpretation is tedious, and its efficacy depends on the skills of expert physicians. In this thesis, we investigate machine learning solutions to promote reliable computer-assisted echo examination, improving interpretation accuracy, diagnostic throughput, and test-retest reliability. The main tackled challenges include considering temporal data dependencies, label sparsity, multi-task learning, low-quality noisy nature of echo, and noisy clinical labels with high inter- and intra-observer variability. We present a deep spatio-temporal model integrating recurrent fully convolutional neural networks and optical flow estimation maps to accurately track the left ventricle in echo video clips. We also propose a semi-supervised learning algorithm to leverage unlabeled data to improve the performance of machine learning methods. Moreover, we present a computationally efficient mobile framework for accurate left ventricular ejection fraction estimation. The proposed mobile application runs in real time on an Android smartphone with a connection to a POCUS or cart-based ultrasound device. We also suggest adapting conditional generative adversarial network (cGAN) architectures to improve the quality of echo data. Further, we investigate predictive uncertainties via Bayesian deep learning to sustain the robust deployment of the developed methodologies.
View record
Prostate cancer is the second most prevalent cancer in men worldwide. Magnetic Resonance Imaging (MRI) is widely used for prostate cancer diagnosis and guiding biopsy procedures due to its ability in providing superior contrast between cancer and adjacent soft tissue. Appropriate clinical management of prostate cancer critically depends on meticulous detection and characterization of the disease and precise biopsy procedures if necessary. The goal of this thesis is to develop computational methods to aid radiologists in diagnosing prostate cancer in MRI and planning necessary interventions. To this end, we have developed novel methods for assessing probability of clinically significant prostate cancer in MRI, localizing biopsy needles in MRI, and providing segmentation of structures such as the prostate gland.The proposed methods in this thesis are based on supervised machine learning techniques, in particular deep convolutional neural networks (CNNs). We have also developed methodology that is necessary in order for such deep networks to eventually be useful in clinical decision-making workflows; this spans the areas of domain adaptation, confidence calibration, and uncertainty estimation for CNNs. We used domain adaptation to transfer the knowledge of lesion segmentation learned from MRI images obtained using one set of acquisition parameters to another. We also studied predictive uncertainty in the context of medical image segmentation to provide model confidence (i.e expectation of success) at inference time. We further proposed parameter ensembling by perturbation for calibration of neural networks.
View record
Biomechanical modelling has the potential to start the next revolution in medicine, just as imaging has done in decades past. Current technology can now capture extremely detailed information about the structure of the human body. The next step is to consider function. Unfortunately, though there have been recent advances in creating useful anatomical models, there are still significant barriers preventing their widespread use.In this work, we aim to address some of the major challenges in biomechanical model construction. We examine issues of discretization: methods for representing complex soft tissue structures; issues related to consolidation of data: how to register information from multiple sources, particularly when some aspects are unreliable; and issues of detail: how to incorporate information necessary for reproducing function while balancing computational efficiency.To tackle discretization, we develop a novel hex-dominant meshing approach that allows for quality control. Our pattern-base tetrahedral recombination algorithm is extremely simple, and has tight computational bounds. We also compare a set of non-traditional alternatives in the context of muscle simulation to determine when each might be appropriate for a given application.For the fusion of data, we introduce a dynamics-driven registration technique which is robust to noise and unreliable information. It allows us to encode both physical and statistical priors, which we show can reduce error compared to the existing methods. We apply this to image registration for prostate interventions, where only parts of the anatomy are visible in images, as well as in creating a subject-specific model of the arm, where we need to adjust for both changes in shape and in pose.Finally, we examine the importance of and methods to include architectural details in a model, such as muscle fibre distribution, the stiffness of thin tendinous structures, and missing surface information. We examine the simulation of muscle contractions in the forearm, force transmission in the masseter, and dynamic motion in the upper airway to support swallowing and speech simulations.By overcoming some of these obstacles in biomechanical modelling, we hope to make it more accessible and practical for both research and clinical use.
View record
The ultimate diagnosis of prostate cancer involves histopathology analysis of tissue samples obtained through prostate biopsy, guided by either transrectal ultrasound (TRUS), or fusion of TRUS with multi-parametric magnetic resonance imaging. Appropriate clinical management of prostate cancer requires accurate detection and assessment of the grade of the disease and its extent. Despite recent advancements in prostate cancer diagnosis, accurate characterization of aggressive lesions from indolent ones is an open problem and requires refinement.Temporal Enhanced Ultrasound (TeUS) has been proposed as a new paradigm for tissue characterization. TeUS involves analysis of a sequence of ultrasound radio frequency (RF) or Brightness (B)-mode data using a machine learning approach. The overarching objective of this dissertation is to improve the accuracy of detecting prostate cancer, specifically the aggressive forms of the disease and to develop a TeUS-augmented prostate biopsy system. Towards full-filling this objective, this dissertation makes the following contributions: 1) Several machine learning techniques are developed and evaluated to automatically analyze the spectral and temporal aspect of backscattered ultrasound signals from the prostate tissue, and to detect the presence of cancer; 2) a patient-specific biopsy targeting approach is proposed that displays near real-time cancer likelihood maps on B-mode ultrasound images augmenting their information; and 3) the latent representations of TeUS, as learned by the proposed machine learning models, are investigated to derive insights about tissue dependent features residing in TeUS and their physical interpretation.A data set consisting of biopsy targets in mp-MRI-TRUS fusion-biopsies with 255 biopsy cores from 157 subjects was used to generate and evaluate the proposed techniques. Clinical histopathology of the biopsy cores was used as the gold-standard. Results demonstrated that TeUS is effective in differentiating aggressive prostate from clinically less-significant disease and non-cancerous tissue. Evidence derived from simulation and latent-feature visualization showed that micro-vibrations of tissue microstructure, captured by low-frequency spectral features of TeUS, is a main source of tissue-specific information that can be used for detection of prostate cancer.
View record
Visualizing vertebrae or other bone structures clearly in ultrasound imaging is important for many clinical applications such as ultrasound-guided spinal needle injections and scoliosis detection. Another growing research topic is fusing ultrasound with other imaging modalities to get the benefit from each modality. In such approaches, tissue with strong interfaces, such as bones, are typically extracted and used as the feature for registration. Among those applications, the spine is of particular interest in this thesis. Although such ultrasound applications are promising, clear visualization of spine structures in ultrasound imaging is difficult due to factors such as specular reflection, off-axis energy and reverberation artifacts. The received channel ultrasound data from the spine are often tilted even after delay correction, resulting in signal cancellation during the beamforming process. Conventional beamformers are not designed to tackle this issue. In this thesis, we propose three beamforming methods dedicated to improve the visualization of spine structures. These methods include an adaptive beamforming method which utilizes the accumulated phase change across the receive aperture as the beamforming weight. Then, we propose a log-Gabor based directional filtering method to regulate the tilted channel data back to the beamforming direction to avoid bone signal cancellation. Finally, we present a closed-loop beamforming method which feeds back the location of the spine to the beamforming process so that backscattered bone signals can be aligned prior-to the beamforming. Field II simulation, phantom and in vivo results confirm significant contrast improvement of spinal structures compared with the conventional delay-and-sum beamforming and other adaptive beamforming methods.
View record
Scaphoid fracture is the most probable outcome of wrist injury and it often occurs due to sudden fall on an outstretched arm. To fix an acute non-displaced fracture, a volar percutaneous surgical procedure is highly recommended as it provides faster healing and better biomechanical outcome to the recovered wrist. Conventionally, this surgical procedure is performed under X-ray based fluoroscopic guidance, where surgeons need to mentally determine a trajectory of the drilling path based on a series of 2D projection images. In addition to challenges associated with mapping 2D information to a 3D space, the process involves exposure to ionizing radiation. Ultrasound (US) has been suggested as an alternate; US has many advantages including its non-ionizing nature and real-time 3D acquisition capability. US images are, however, difficult to interpret as they are often corrupted by significant amounts of noise or artifact, in addition, the appearance of the bone surfaces in an US image contains only a limited view of the true surfaces. In this thesis, I propose techniques to enable ultrasound guidance in scaphoid fracture fixation by augmenting intraoperative US images with preoperative computed tomography (CT) images via a statistical anatomical model of the wrist. One of the major contributions is the development of a multi-object statistical wrist shape+scale+pose model from a group of subjects at wide range of wrist positions. The developed model is then used to register with the preoperative CT to obtain the shapes and sizes of the wrist bones. The intraoperative procedure starts with a novel US bone enhancement technique that takes advantage of an adaptive wavelet filter bank to accurately highlight the bone responses in US. The improved bone enhancement in turn enables a registration of the statistical pose model to intraoperative US to estimate the optimal scaphoid screw axis for guiding the surgical procedure. In addition to this sequential registration technique, I propose a joint registration technique that allows a simultaneous fusion of the US and CT data for an improved registration output. We conduct a cadaver experiment to determine the accuracy of the registration process, and compare the results with the ground truth.
View record
Low-dose-rate prostate brachytherapy is a minimally invasive treatment approach for localized prostate cancer. It takes place in one session by permanent implantation of several small radio-active seeds inside and adjacent to the prostate. The current procedure at the majority of institutions requires planning of seed locations prior to implantation from transrectal ultrasound (TRUS) images acquired weeks in advance. The planning is based on a set of contours representing the clinical target volume (CTV). Seeds are manually placed with respect to a planning target volume (PTV), which is an anisotropic dilation of the CTV, followed by dosimetry analysis. The main objective of the plan is to meet clinical guidelines in terms of recommended dosimetry by covering the entire PTV with the placement of seeds. The current planning process is manual, hence highly subjective, and can potentially contribute to the rate and type of treatment related morbidity. The goal of this thesis is to reduce subjectivity in prostate brachytherapy planning. To this end, we developed and evaluated several frameworks to automate various components of the current prostate brachytherapy planning process. This involved development of techniques with which target volume labels can be automatically delineated from TRUS images. A seed arrangement planning approach was developed by distributing seeds with respect to priors and optimizing the arrangement according to the clinical guidelines. The design of the proposed frameworks involved the introduction and assessment of data fusion techniques that aim to extract joint information in retrospective clinical plans, containing the TRUS volume, the CTV, the PTV and the seed arrangement. We evaluated the proposed techniques using data obtained in a cohort of 590 brachytherapy treatment cases from the Vancouver Cancer Centre, and compare the automation results with the clinical gold-standards and previously delivered plans. Our results demonstrate that data fusion techniques have the potential to enable automatic planning of prostate brachytherapy.
View record
Prostate biopsy is the gold standard for cancer diagnosis. This procedure is guided using a 2D transrectal ultrasound (TRUS) probe. Unfortunately, early stage tumors are not visible in ultrasound and prostate motion/deformations make targeting challenging. This results in a high number of false negatives and patients are often required to repeat the procedure. Fusion of magnetic resonance images (MRI) into the workspace of a prostate biopsy has the potential to detect tumors invisible in TRUS. This allows the radiologist to better target early stage cancerous lesions. However, due to different body positions and imaging settings, the prostate undergoes motion and deformation between the biopsy coordinate system and the MRI. Furthermore, due to variable probe pressure, the prostate moves and deforms during biopsy as well. This introduces additional targeting errors. A biopsy system that compensates for these sources of error has the potential to improve the targeting accuracy and maintain a 3D record of biopsy locations. The goal of this thesis is to provide the necessary tools to perform freehand MR-TRUS fusion for prostate biopsy using a 3D guidance system. To this end, we have developed two novel surface-based registration methods for incorporating the MRI into the biopsy workspace. The proposed methods are the first methods that are robust to missing surface regions for MR-TRUS fusion (up to 30% missing surface points). We have validated these fusion techniques on 19 biopsy, 10 prostatectomy and 11 brachytherapy patients. In this thesis, we have also developed methods that combine intensitybased information with biomechanical constraints to compensate for prostate motion and deformations during the biopsy. To this end, we have developed a novel 2D-3D registration framework, which was validated on an additional 10 biopsy patients. Our results suggest that accurate 2D-3D registration for freehand biopsy is feasible.The results presented suggest that accurate registration of MR and TRUS data in the presence of partially missing data is feasible. Moreover, we demonstrate that in the presence of variable probe pressure during freehand biopsy, a combination of intensity-based and biomechanically constrained 2D-3D registration can enable accurate alignment of pre-procedure TRUS with 2D real time TRUS images.
View record
Diagnosis and clinical management of neurological disorders that affect brain structure, function and networks would benefit substantially from the development of techniques that combine multi-modal and/or multi-task information. Here, we propose a joint Source Based Analysis (jSBA) framework to identify common information across structural and functional contrasts in data from MRI and fMRI experiments, for classification of individuals with neurological and psychiatric disorders. The framework consists of three components: 1) individual's feature generation, 2) joint group analysis, and 3) classification of individuals based on the group's generated features. In the proposed framework, information from brain neuroimaging datasets is reduced to a feature that is a lower-dimensional representation of a selected brain structure or task-related activation pattern. For each individual, features are used within a joint analysis method to generate basis brain activation sources and their corresponding modulation profiles. Modulation profiles are used to classify individuals into different categories. We perform two experiments to demonstrate the potential of the proposed framework to classify groups of subjects based on structural and functional brain data. In the fMRI analysis, functional contrast images derived from a study of auditory and speech perception of 16 young and 16 older adults are used for classification of individuals. First, we investigate the effect of using multi-task fMRI data to improve the classification accuracy. Then, we propose a novel joint Sparse Representation Analysis (jSRA) to identify common information across different functional contrasts in data. We further assess the reliability of jSRA, and visualize the brain patterns obtained from such analysis. In the sMRI analysis, features representing position, orientation and size (i.e. pose), shape, and local tissue composition of brain are used to classify 19 depressed and 26 healthy individuals. First, we incorporate pose and shape measures of morphology, which are not usually analyzed in neuromorphometric studies, to measure structural changes. Then, we combine brain tissue composition and morphometry using the proposed jSBA framework. In a cross-validation leave-one-out experiment, we show that we can classify the subjects with an accuracy of 67% solely based on the information gathered from the joint analysis of features obtained from multiple brain structures.
View record
Ultrasound is a safe, portable, inexpensive and real-time modality that can produce 2D and 3D images. It is a valuable intra-operative imaging modality to guide surgeons aiming to achieve higher accuracy of the intervention and improve patient outcomes. In all the clinical applications that use tracked ultrasound, one main challenge is to precisely locate the ultrasound image pixels with respect to a tracking sensor on the transducer. This process is called spatial calibration and the objective is to determine the spatial transformation between the ultrasound image coordinates and a coordinate system defined by the tracking sensor on the transducer housing. Another issue in ultrasound guided interventions is that tracking surgical tools (for example an epidural needle) usually requires expensive, large optical trackers or low accuracy magnetic trackers and there is a need for a low-cost, easy-to-use and accurate solution. In this thesis, for the first problem I have proposed two novel complementary methods for ultrasound calibration that provide ease of use and high accuracy. These methods are based on my differential technique which enables high measurement accuracy. I developed a closed-form formulation that makes it possible to achieve high accuracy with using a low number of images. For the second problem, I developed a method to track surgical tools (epidural needles in particular) using a single camera mounted on the ultrasound transducer to facilitate ultrasound guided interventions. The first proposed ultrasound calibration method achieved an accuracy of 0.09 ± 0.39 mm. The second method with a much simpler phantom yet achieved similar accuracy compared to the N-wire method. The proposed needle tracking method showed high accuracy of 0.94 ± 0.46 mm.
View record
The idea of full six degree-of-freedom tracking of ultrasound images solely based on speckle information has been a long term research goal. It would eliminate the need for any additional tracking hardware and reduces cost and complexity of ultrasound imaging system, while providing the benefits of three-dimensional imaging.Despite its significant promise, speckle tracking has proven challenging due to several reasons including the dependency on a rare kind of speckle pattern in real tissue, underestimation in the presence of coherency or specular reflection, ultrasound beam profile spatial variations, need for RF (Radio Frequency) data, and artifacts produced by out-of-plane rotation. So, there is a need to improve the utility of freehand ultrasound in clinics by developing techniques to tackle these challenges and evaluate the applicability of the proposed methods for clinical use.We introduce a model-fitting method of speckle tracking based on the Rician Inverse Gaussian (RiIG) distribution. We derive a closed-form solution of the correlation coefficient of such a model, necessary for speckle tracking. In this manner, it is possible to separate the effect of the coherent and the non-coherent part of each patch. We show that this will increase the accuracy of the out-of-plane motion estimation.We also propose a regression-based model to compensate for the spatial changes of the beam profile.Although RiIG model fitting increases the accuracy, it is only applicable on ultrasound sampled RF data and computationally expensive. We propose a new framework to extract speckle/noise directly from B-mode images and perform speckle tracking on the extracted noise. To this end, we investigate and develop Non-Local Means (NLM) denoising algorithm based on a prior noise formation model.Finally, in order to increase the accuracy of the 6-DoF transform estimation, we propose a new iterative NLM denoising filter for the previously introduced RiIG model based on a new NLM similarity measure definition. The local estimation of the displacements are aggregated using Stein’s Unbiased Risk Estimate (SURE) over the entire image. The proposed filter-based speckle tracking algorithm has been evaluated in a set of ex vivo and in vivo experiments.
View record
The blind placement of an epidural needle is among the most difficult regional anesthetic techniques. The challenge is to insert the needle in the midline plane of the spine and to avoid overshooting the needle into the spinal cord. Prepuncture 2D ultrasound scanning has been introduced as a reliable tool to localize the target and facilitate epidural needle placement. Ideally, real-time ultrasound should be used during needle insertion to monitor the progress of needle towards the target epidural space. However, several issues inhibit the use of standard 2D ultrasound, including the obstruction of the puncture site by the ultrasound probe, low visibility of the target in ultrasound images of the midline plane, and increased pain due to a longer needle trajectory. An alternative is to use 3D ultrasound imaging, where the needle and target could be visible within the same reslice of a 3D volume; however, novice ultrasound users (i.e., many anesthesiologists) have difficulty interpreting ultrasound images of the spine and identifying the target epidural space. In this thesis, I propose techniques that are utilized for augmentation of 3D ultrasound images with a model of the vertebral column. Such models can be pre-operatively generated by extracting the vertebrae from various imaging modalities such as Computed Tomography (CT) or Magnetic Resonance Imaging (MRI). However, these images may not be obtainable (such as in obstetrics), or involve ionizing radiation. Hence, the use of Statistical Shape Models (SSM) of the vertebrae is a reasonable alternative to pre-operative images. My techniques include construction of a statistical model of vertebrae and its registration to ultrasound images. The model is validated against CT images of 56 patients by evaluating the registration accuracy. The feasibility of the model is also demonstrated via registration to 64 in vivo ultrasound volumes.
View record
Theses completed in 2010 or later are listed below. Please note that there is a 6-12 month delay to add the latest theses.
Aortic stenosis (AS) is a valvular cardiac disease that results in restricted motion and calcification of the aortic valve (AV). AS severity is currently assessed by expert cardiologists using Doppler measurements from echocardiography (echo). However, this method limits the assessment of AS to hospitals with access to expert cardiologists and comprehensive echo service. This thesis explores the feasibility of using deep neural network (DNN) for AS detection and severity classification based solely on two-dimensional echocardiographic data. While several machine learning (ML) frameworks have been developed for echo diagnosis and other medical applications, most of them rely on black-box models with low trustworthiness, and they cannot be trained effectively due to the scarcity of training data. However, a model’s explanability and generalizability are essential for clinician adoption. Therefore, a model should be able to capture critical information in both spatial and temporal dimensions of echo videos and provide explanations to support its decision. This thesis proposes frameworks that enhance the state-of-the-art by offering explanability and accurate assessment from echo videos, making ML more practical in echo examination. The first proposed framework aims to compare well-known video models in ML literature for detection and severity assessment of AS. In addition, we leverage semi-supervised learning to fine-tune model weights with unlabeled data. In the second framework, we propose a spatio-temporal architecture that effectively combines both anatomical features and motion of the AV for AS severity classification. Our model can identify the frames that are most informative towards the AS diagnosis and learns phases of the heart cycle without any supervision and frame-level annotations. Furthermore, our method addresses common problems in training deep networks with clinical ultrasound data, such as a low signal-to-noise ratio and frequently uninformative frames. Finally, we address the issue of explanability by incorporating two prototypical layers into existing architectures, enabling interpretable predictions based on the similarity between input and learned prototypes. This approach offers clinically relevant evidence by highlighting markers like calcification and restricted movement of AV leaflets, aiding in more accurate screening examinations.
View record
Standard deep learning paradigm may not be practical over real-world heterogeneous medical data, where new disease merges over time with data acquired in a distributed manner across various hospitals. There have been approaches to facilitate the training of deep models over two primary categories of heterogeneity, including 1) class incremental learning, which offers a promising solution for sequential heterogeneity by adapting a deep network trained on previous disease classes to handle newly introduced diseases over time; 2) federated learning, which offers a promising solution for distributed heterogeneity, by training a global model on a centralized server over private datasets of various hospitals or clients without requiring them to share data. The core challenge in both approaches is catastrophic forgetting, which refers to performance degradation on previously trained data when adapting a model to available data. Due to strict patient privacy regulations, storing and sharing medical data are often discouraged, posing a significant hurdle in addressing such a forgetting. We propose to leverage medical data synthesis to recover inaccessible medical data in heterogeneous learning, presenting two distinctive novel frameworks. Our first framework introduces a novel two-step, data-free class incremental learning pipeline. Initially, it synthesizes data by inverting trained model weights on previous classes and matching statistics saved in continual normalization layers to obtain continual class-specific samples. Subsequently, the model is updated by incorporating three novel loss functions to enhance the utility of synthesized data and mitigate forgetting. Extensive experiments demonstrate that the proposed framework achieves comparative results with state-of-the-art methods on four public MedMNIST datasets and an in-house heart echocardiography dataset. We propose our second framework as a novel federated learning approach to mitigate forgetting by generating and utilizing united global synthetic data among clients. First, we proposed constrained model inversion over the server model to enforce an information-preserving property in synthetic data and leverage the global distribution captured in the globally aggregated server model. Then, we utilize this synthetic data alongside the local data to enhance the generalization capabilities of local training. Extensive experiments show that the proposed method achieves state-of-the-art performance on the BloodMNIST and Retina datasets.
View record
Background: In contrast to histopathological assessment, molecular subtyping of Endometrial Cancer (EC) provides a reproducible classification system with significant prognostic value. Proactive Molecular Risk Classifier for Endometrial Cancer (ProMisE) was developed as a practical, cost-efficient, and therapeutically beneficial molecular classifier, replacing complex genomic tests. ProMisE stratifies EC into four subtypes: (1) POLE mutant, (2) Mismatch repair deficient, (3) p53 abnormal (p53abn) by immunohistochemistry, and (4) No Specific Molecular Profile (NSMP), which lacks any of the distinguishing features of the other three subtypes. Although ProMisE has provided significant prognostic value, there are clinical outliers within its four subtypes. This is especially evident in the largest ProMisE subtype, NSMP, accounting for about half of all ECs, where a fraction of patients encounter a very aggressive disease course, similar to the behavior of patients diagnosed with p53abn. Method: We considered the problem of refining the EC NSMP subtype using ubiquitous histopathology images. We hypothesized that evaluating the digital hematoxylin and eosin-stained images of NSMP could discern clinical outcome outliers. To this end, we designed an image analysis framework utilizing Artificial Intelligence (AI) to detect NSMP patients with comparable histological characteristics to the p53abn subtype. The analysis included various preprocessing steps, deep neural networks classifying the subtype of images, and survival and genomic analyses. Finding: Exploiting an AI-based methodology, we have expanded the NSMP subtype into two subgroups: ‘p53abn-like’ NSMPs and the rest of the NSMP cases. The former consists of patients diagnosed with NSMP by ProMisE, yet our AI-based analysis labeled them as p53abn due to morphological similarities. With following similar trends in two independent datasets, ‘p53abn-like’ NSMPs displayed comparable clinical behavior to p53abn, where they had markedly unfavorable outcomes in comparison with the remainder of the NSMP cases. In addition, the extensive genomic analysis suggested that ‘p53abn- like’ NSMPs had significantly higher fractions of genome altered than NSMPs in both datasets, validating our initial hypothesis in a different domain of data. We also discovered that ‘p53abn-like’ NSMPs patients might not benefit from hormone therapy. These findings emphasize the potential of AI screening as a stratification tool within ProMisE.
View record
Supervised machine learning is the standard workflow in training state-of-the-art deep neural networks to automatically analyze, classify, or quantify ultrasound images or videos. However, there are certain challenges regarding the available label set in this context. Since expert annotation is a tedious process, fine-grained and extensive labels are usually not available. The size of data is often limited, and missing labels are an issue. Due to observer variability among different annotators, there is variability in ground truth that manifests as a type of label noise. This thesis aims to investigate the use of multi-task learning to alleviate these issues, in the context of two problems. The first problem is echocardiographic video landmark detection, to quantify the dimensions of the left ventricle of the heart. We first propose a two-headed U-net-shaped convolutional neural network, to detect pairs of inner and outer landmarks on the left ventricle. The model is weakly supervised on the two frames of the video with annotation, with the outer landmarks missing at one of them. Secondly, we propose Differential Learning, which adds a task of ejection fraction comparison to the landmark detection framework, in a Siamese architecture that is trained end-to-end with the main tasks. This auxiliary task is designed to have very low observer noise, by comparing samples that have sufficiently different ejection fractions. We show that this multi-headed model overcomes the issue of missing labels, and Differential Learning improves the results by providing a less noisy training signal. The second problem is biomarker detection, and disease classification in lung ultrasound videos, to detect Covid-19 infection. A multi-headed attention model is first proposed to detect lung biomarkers (A-lines and B-lines) that appear sporadically in lung ultrasound videos, trained on a private and limited dataset with coarse video-level labels. We then propose knowledge transfer to fine-tune this network on the disease classification task in a public lung ultrasound video dataset. We validate this method's ability to overcome limitations in data and label, through ablation studies and comparison to the state-of-the-art. Our proposed attention-scaled explainability method also visualizes the model’s attention to clinically relevant features.
View record
Background: In comparison with other advanced imaging techniques (e.g. computed tomography, or magnetic resonance imaging), cardiac ultrasound interpretation is less accurate with higher prevalence of low quality images. The problem can be more severe when non-experts use point-of-care ultrasound (PoCUS) to acquire and interpret images. Artificial intelligence (AI) models that provide image quality rating and feedback can help novice users to identify suboptimal image quality in real-time. However, such models have only been validated on cart-based ultrasound systems typically used in echocardiography labs. In this study, we examined the performance of a AI deep learning image quality feedback model trained on cart-based ultrasound systems when applied to PoCUS devices. Methods: We enrolled 107 unselected patients from an out-patient echocardiography facility at the Vancouver General Hospital. A single sonographer obtained 9 standard image views with a cart-based system and with a hand-held PoCUS device. All the images obtained were assigned image quality ratings by the AI model and by 2 expert physician echocardiographers. Image quality was graded based on percent endocardial border visualization (poor quality = 0-25%; fair quality = 26-50%; good quality = 51-75%; excellent quality = 76-100%). Statistical methods were used to compare the model’s classification performance on cart-based vs. PoCUS data with respect to echocardiographer opinion: percent agreement, weighted kappa, positive predictive value (precision), negative predictive value, sensitivity (recall), and specificity. Results: Percent agreement and weighted kappa were comparable on PoCUS and cart-based ultrasound clips. Overall, the model’s positive predictive value, negative predictive value, sensitivity, and specificity were neither better nor worse on either machine type. Conclusions: We conclude that AI based image quality feedback models designed for cart-based systems can perform well when applied to hand-held PoCUS devices. Researchers may consider using cart-based ultrasound data to train models for PoCUS to overcome data collection and labelling barriers.
View record
Ejection fraction is a widely-used and critical index of cardiac health. It measures the efficacy of the cyclic contraction of the ventricles and the outward pumpage of blood through the arteries.Timely and robust evaluation of ejection fraction is essential, as reduced ejection fraction indicates dysfunction in blood delivery during the ventricular systole, and is associated with a number of cardiac and non-cardiac risk factors and mortality-related outcomes. Automated reliable ejection fraction estimation in echocardiography has proven challenging due to low and variable image quality, and limited amounts of data for training data-driven algorithms, which delays the integration of the technologies in the clinical workflow. Deep learning has shown state-of-the-art performance in many learning tasks especially in learning from image and video datasets. While deep learning models give promising results in these fields, they are usually over-confident about their outputs and predictions. However, in many applications like the ones related to human health and safety, a well-calibrated and reliable uncertainty estimation is required. In this thesis, we review the most important results in the literature of uncertainty estimation in deep learning and then, propose multiple Bayesian and non-Bayesian deep models to estimate the ejection fraction from echocardiography data along with the epistemic and aleatoric uncertainties associated with these estimations. Finally, we evaluate these models by training and testing them on a publicly available dataset, and by making a side-by-side comparison of them with their deterministic counterparts. Our results show the feasibility of those methods to be deployed in healthcare applications. Also based on the presented rationale and results, we believe that the proposed approach can further be thought of as a generic approach for a more robust evaluation of critical clinical indices.
View record
The length of the left ventricle and how it changes throughout a cardiac cycle is an indicative of the health of the heart in clinical studies. Currently, this measurement is computed through manual labeling made by experienced sonographers. This labeling procedure is highly costly, time-consuming, and prone to inter-observer and intra-observer errors. In order to overcome these challenges, this thesis proposes a deep neural network-based model to automate the detection of landmarks corresponding to the diameter of the heart. The dataset used for this work is sparse in the temporal dimension, having annotations merely available on end-diastolic and end-systolic frames, and the final goal of the model is to provide meaningful measurements across the entire cardiac cycle. The proposed network leads to 10.08% average ejection fraction error, and 12.18% mean percentile error, which is satisfactory based on the requirements of this project.
View record
Coronary Artery Disease (CAD) is the leading cause of morbidity and mortality in developed nations. In patients with acute or chronic obstructive CAD, Echocardiography (ECHO) is the standard-of-care for visualizing abnormal ventricular wall thickening or motion which would be reported as Regional WallMotion Abnormality (RWMA). The accurate identification of regional wall motion abnormalities is essential for cardiovascular assessment and myocardial ischemia, coronary artery disease and myocardial infarction diagnosis. Given the variability and challenges of scoring regional wall motion abnormalities, we propose the development of a platform that can quickly and accurately identify regional and global wall motion abnormalities on echo images. This thesis describes a deep learning-based framework that can aid physicians to utilize ultrasound for wall motion abnormality detection. The framework jointly combines image data and patient diagnostic information to determine both global and clinically-standard 16 regional wall motion labels. We validate the approach on a large cohort of echo studies obtained from 953 patients. We then report the performance of the proposed framework in the detection of wall motion abnormality. An average accuracy of 69.2% for the 16 regions and an average accuracy of 69.5% for global wall motion abnormality were achieved. To the best of our knowledge, our proposed framework is the first to analyze left ventricle wall motion for both global and regional abnormality detection in echocardiography data.
View record
Magnetic Resonance (MR) and Computed Topography (CT) are the most common modalities for spine imaging. Localization and identification of vertebrae is an essential first step in examining these volumes for diagnosis, surgical planning and management of patients with disc or vertebra pathologies and conditions. With large volumes of spinal scans acquired at imaging centres, development of a computerized solution for spine labelling has received attention from several research groups, as it can help save radiologists time and clicks. It can also expedite the imaging-dependent pre- and post-operation procedures. Nonetheless, automatic spine labelling in CT and MR is non-trivial and has proven challenging. This is due to: 1) limited and variable field-of-view (FOV); 2) variability in imaging parameters and resolution; 3) variability in shape, size and appearance of the spinal anatomies, especially in the presence of various pathologies or implants; 4) the repetitive nature of the spine and similar appearance of the vertebrae; and particularly for learning-based solutions, 5) dependence on expert annotations. In this thesis, learning-based approaches that perform simultaneous identification and localization of vertebrae are introduced. The principal goal is to design a supervised spine labelling approach that requires minimal manual annotations, and can perform both identification and localization tasks within a unified framework. We achieved an identification rate of 89.76%.
View record
Spinal needle procedures require identification of the vertebral level for effectiveness and safety. E.g. in obstetric epidurals, the preferred target is between the third and fourth lumbar vertebra. The current clinical standard involves "blind" identification of the level through manual palpation, which only has a 30% reported accuracy. Therefore, there is a need for better anatomical identification prior to needle insertion. Ultrasound provides anatomical information to physicians, which is not obtainable via manual palpation. However, due to artifacts and the complex anatomy of the spine, ultrasound is not commonly used for pre-puncture planning.This thesis describes two machine learning based systems that can aid physicians to utilize ultrasound for lumbar level identification.The first system, LIT, is proposed to identify vertebrae, assigning them to their respective levels and tracking them in a sequence of ultrasound images in the paramedian plane. A deep sparse auto-encoder network learns to extract anatomical features from pre-processed ultrasound images. A feasibility study (n=15) evaluated performance.The second system, SLIDE, identifies vertebral levels from a sequence of ultrasound images in the transverse plane. The system uses a deep convolutional neural network (CNN) to classify transverse planes of the lower spine. In conjunction, a novel state-machine is developed to automatically identify vertebral levels as the transducer moves.A feasibility study (n=20) evaluated performance. The CNN achieves 88% accuracy in discriminating images from three planes of the spine. As a system, SLIDE successfully identifies all lumbar levels in 17 of 20 test scans, processed at real-time speed.A clinical study with 76 parturient patients was performed. The study compares level identification accuracy between manual palpation, versus SLIDE, with both compared to freehand ultrasound. SLIDE's level identification outperformed palpation with an odds ratio of nearly 3. A subset of recorded ultrasound (n=60) was labeled and used to retrain the CNN, improving classification accuracy to 93%.The systems showcase the utility of machine learning in spinal ultrasound analysis, with varied approaches to automatically identifying vertebral levels. The systems can be used to improve the accuracy of vertebral level identification compared to manual palpation alone.
View record
Facet joint injections and epidural needle insertions are widely used for spine anesthesia. Needle guidance is usually performed by fluoroscopy or palpation, resulting in radiation exposure and multiple needle re-insertions. Several ultrasound (US)-based guidance approaches have been proposed to eliminate such issues.However, but they have not widely accepted in clinics due to difficulties in interpretation of the complex spinal anatomy in US, which leads to clinicians' lack of confidence in relying only on information derived from US for needle guidance.In this thesis, a model-based multi-modal joint registration framework is introduced, where a statistical model of the lumbar spine is concurrently registered to intraprocedure US and easy-to-interpret preprocedure images. The goal is to take advantage of the complementary features visible in US and preprocedure images, namely Computed Topography (CT) and Magnetic Resonance (MR) scans. Two versions of a lumbar spine statistical model are employed: a shape+pose model and a shape+pose+scale model. The underlying assumption is that the shape and size of the spine of a given subject are common amongst all imaging modalities . However, the pose of the spine changes from one modality to another, as the patient's position is different at different image acquisitions. The proposed method has been successfully validated on two datasets: (i) 10 pairs of US and CT scans and (ii) nine US and MR images of the lumbar spine. Using the shape+pose+scale model on the US+CT dataset, mean surface distance error of 2.42 mm for CT and mean Target Registration Error (TRE) of 3.14 mm for US were achieved. As for the US+MR dataset, TRE of 2.62 mm and 4.20 mm for the MR and US images, respectively. Both models models were equally accurate on the US+CT dataset. For US+MR, the shape+pose+scale model outperformed the shape+pose model. The joint registration allows augmentation of important anatomical landmarks in both intraprocedure US and preprocedure domains. Furthermore, observing the patient-specific model in preprocedure domains allows the clinicians to assess the local registration accuracy qualitatively. This can increase their confidence in using the US model for deriving needle guidance decisions.
View record
We propose a joint information framework for automatic analysis of 2D echocardiography (echo) data. The analysis combines a priori images, their segmentations and patient diagnostic information within a unified framework to determine various clinical parameters, such as cardiac chamber volumes, and cardiac disease labels. The main idea behind the framework is to employ joint Independent Component Analysis of both echo image intensity information and corresponding segmentation labels to generate models that jointly describe the image and label space of echo patients on multiple apical views jointly, instead of independently. These models are then both used for segmentation and volume estimation of cardiac chambers such as the left atrium and for detecting pathological abnormalities such as mitral regurgitation. We validate the approach on a large cohort of echos obtained from 6,993 studies. We report performance of the proposed framework in estimation of the left-atrium volume and diagnosis of mitral-regurgitation severity. A correlation coefficient of 0.87 was achieved for volume estimation of the left atrium when compared to the clinical report. Moreover, we classified patients that suffer from moderate or severe mitral regurgitation diagnosis with an average accuracy of 82%. Using only B-Mode echo information to automatically derive these clinical parameters, there is potential for this approach to be used clinically.
View record
Automatic localization and identification of vertebrae in medical images of the spine are core requirements for building computer-aided systems for spine diagnosis. Automated algorithms for segmentation of vertebral structures can also benefit these systems for diagnosis of a range of spine pathologies. The fundamental challenges associated with the above-stated tasks arise from the repetitive nature of vertebral structures, restrictions in field of view, presence of spine pathologies or surgical implants, and poor contrast of the target structures in some imaging modalities. This thesis presents an automatic method for localization, identification, and segmentation of vertebrae in volumetric computed tomography (CT) scans and magnetic resonance (MR) images of the spine. The method makes no assumptions about which section of the vertebral column is visible in the image. An efficient deep learning approach is used to predict the location of each vertebra based on its contextual information in the image. Then, a statistical multi-vertebrae model is initialized by the localized vertebrae from the previous step. An iterative expectation maximization technique is used to register the statistical multi-vertebrae model to the edge points of the image in order to achieve a fast and reliable segmentation of vertebral bodies. State-of-the-art results are obtained for vertebrae localization in a public dataset of 224 arbitrary-field-of-view CT scans of pathological cases. Promising results are also obtained from quantitative evaluation of the automated segmentation method on volumetric MR images of the spine.
View record
We propose an augmented reality system to automatically identify lumbar vertebral levels and the lamina region in ultrasound-guided epidural anesthesia. Spinal needle insertion procedures require careful placement of a needle, both to ensure effective therapy delivery and to avoid damaging sensitive tissue such as the spinal cord. An important step in such procedures is the accurate identification of the vertebral levels, which is currently performed using manual palpation with a reported success rate of only 30%. In this thesis, we propose a system using a trinocular camera which tracks an ultrasound transducer during the acquisition of a sequence of B-mode images. The system generates a panorama ultrasound image of the lumbar spine, automatically identifies the lumbar levels in the panorama image, and overlays the identified levels on a live camera view of the patient’s back. Several experiments were performed to test the accuracy of vertebral height in panorama images, the accuracy of vertebral levels identification in panorama images, the accuracy of vertebral levels identification on the skin, and the impact on accuracy with spine arching. The results from 17 subjects demonstrate the feasibility of the approach and capability of achieving an error within a clinically acceptable range for epidural anesthesia. The overlaid marks on the screen are used to assist locating needle puncture site. Then, an automated slice selection algorithm is used to guide the operator positioning a 3D transducer such that the best view of the target anatomy is visible in a predefined re-slice of the 3D ultrasound volume. This re-slice is used to observe, in real time, the trajectory of a needle attached to the 3D transducer, towards the target. The method is based on Haar-like features and AdaBoost learning algorithm. We have evaluated the method on a set of 32 volumes acquired from volunteer subjects by placing the 3D transducer on L1-L2, L2-L3, L3-L4 and L4- L5 interspinous gaps on each side of the lumbar spine. Results show that the needle insertion plane can be identified with a root mean square error of 5.4 mm, accuracy of 99.6%, and precision of 78.7%.
View record
Robotic laparoscopic partial nephrectomy is a state-of-the-art procedure for the excision of renal tumours. The challenges of this surgery along with the stereoscopic interface to the surgeon make it an ideal candidate for image guidance. We propose bringing pre-operative computed tomography data to the patient's coordinate system using three-dimensional intraoperative back ultrasound. Since computed tomography and ultrasound images represent like anatomical information quite differently, we perform a manual segmentation of the computed tomography before the operation and a semi-automatic segmentation of the ultrasound intra-operatively. The segmentation of the kidney boundary facilitates a feature-based registration strategy.Semi-automatic segmentation of kidney ultrasound images is difficult because the edges with large gradient values do not correspond to the capsule boundary seen in computed tomography. The desired edges are actually quite faint in ultrasound and poorly detected by common edge methods such as the Canny approach.After trying a number of approaches, the best results were obtained using a novel interacting multiple-model probabilistic data association filter to select edges from ultrasound images that were filtered for phase congruency. The manual segmentation of the prior is used to guide edge detection in ultrasound. Experiments on seven pre-operative patient datasets and one intra-operative patient dataset resulted in a mean volume error ratio of 0.80 +/- 0.13 from after registration to before registration. These results came after the implementation and evaluation of numerous other approaches, including radial edge filters, the covariance matrix adaptation evolution strategy, and a deformable approach using geodesic active contours.The main contribution of this work is a method for the registration of the pre-operative planning data from computerized tomography to the intraoperative ultrasound. For clinical use, this method requires some form of calibration with the laparoscopic camera and integration with surgical visualization tools. Through integration with emerging technologies, the approach presented here can one day augment the surgical field-of-view and guide the surgeon around important anatomical structures to the tissue that must be excised.
View record
If this is your researcher profile you can log in to the Faculty & Staff portal to update your details and provide recruitment preferences.